Open Access
ARTICLE
Adaptive Error Curve Learning Ensemble Model for Improving Energy Consumption Forecasting
Department of Computer Engineering, Jeju National University, Jeju-si, Korea
* Corresponding Author: Yung-Cheol Byun. Email:
(This article belongs to the Special Issue: Emerging Trends in Artificial Intelligence and Machine Learning)
Computers, Materials & Continua 2021, 69(2), 1893-1913. https://doi.org/10.32604/cmc.2021.018523
Received 10 March 2021; Accepted 11 April 2021; Issue published 21 July 2021
Abstract
Despite the advancement within the last decades in the field of smart grids, energy consumption forecasting utilizing the metrological features is still challenging. This paper proposes a genetic algorithm-based adaptive error curve learning ensemble (GA-ECLE) model. The proposed technique copes with the stochastic variations of improving energy consumption forecasting using a machine learning-based ensembled approach. A modified ensemble model based on a utilizing error of model as a feature is used to improve the forecast accuracy. This approach combines three models, namely CatBoost (CB), Gradient Boost (GB), and Multilayer Perceptron (MLP). The ensembled CB-GB-MLP model’s inner mechanism consists of generating a meta-data from Gradient Boosting and CatBoost models to compute the final predictions using the Multilayer Perceptron network. A genetic algorithm is used to obtain the optimal features to be used for the model. To prove the proposed model’s effectiveness, we have used a four-phase technique using Jeju island’s real energy consumption data. In the first phase, we have obtained the results by applying the CB-GB-MLP model. In the second phase, we have utilized a GA-ensembled model with optimal features. The third phase is for the comparison of the energy forecasting result with the proposed ECL-based model. The fourth stage is the final stage, where we have applied the GA-ECLE model. We obtained a mean absolute error of 3.05, and a root mean square error of 5.05. Extensive experimental results are provided, demonstrating the superiority of the proposed GA-ECLE model over traditional ensemble models.Keywords
Cite This Article
Citations
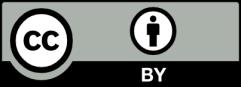