Open Access
ARTICLE
A Hybrid Feature Selection Framework for Predicting Students Performance
1 High Performance Cloud Computing (HPC3), Department of Computer and Information Sciences, Universiti Teknologi PETRONAS, 32610, Seri, Iskandar, Malaysia
2 Department of Computer Science and Information Technology, University of Lahore, Pakistan
3 Department of Process Engineering, Pakistan Institute of Engineering & Applied Sciences, Nilore, Islamabad, Pakistan
4 Electrical Engineering Department, College of Engineering, Najran University, Najran, 61441, Saudi Arabia
5 College of Computer Science and Information Systems, Najran University, 61441, Najran, Saudi Arabia
* Corresponding Author: Maryam Zaffar. Email:
(This article belongs to the Special Issue: Machine Learning Applications in Medical, Finance, Education and Cyber Security)
Computers, Materials & Continua 2022, 70(1), 1893-1920. https://doi.org/10.32604/cmc.2022.018295
Received 04 March 2021; Accepted 24 May 2021; Issue published 07 September 2021
Abstract
Student performance prediction helps the educational stakeholders to take proactive decisions and make interventions, for the improvement of quality of education and to meet the dynamic needs of society. The selection of features for student's performance prediction not only plays significant role in increasing prediction accuracy, but also helps in building the strategic plans for the improvement of students’ academic performance. There are different feature selection algorithms for predicting the performance of students, however the studies reported in the literature claim that there are different pros and cons of existing feature selection algorithms in selection of optimal features. In this paper, a hybrid feature selection framework (using feature-fusion) is designed to identify the significant features and associated features with target class, to predict the performance of students. The main goal of the proposed hybrid feature selection is not only to improve the prediction accuracy, but also to identify optimal features for building productive strategies for the improvement in students’ academic performance. The key difference between proposed hybrid feature selection framework and existing hybrid feature selection framework, is two level feature fusion technique, with the utilization of cosine-based fusion. Whereas, according to the results reported in existing literature, cosine similarity is considered as the best similarity measure among existing similarity measures. The proposed hybrid feature selection is validated on four benchmark datasets with variations in number of features and number of instances. The validated results confirm that the proposed hybrid feature selection framework performs better than the existing hybrid feature selection framework, existing feature selection algorithms in terms of accuracy, f-measure, recall, and precision. Results reported in presented paper show that the proposed approach gives more than 90% accuracy on benchmark dataset that is better than the results of existing approach.Keywords
Cite This Article
Citations
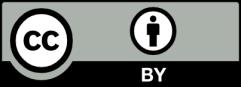