Open Access
ARTICLE
Deep Stacked Ensemble Learning Model for COVID-19 Classification
1 Department of Information Technology, VNRVJIET, Hyderabad, 500090, India
2 Department of Computer Science and Engineering, VNRVJIET, Hyderabad, 500090, India
3 LBEF Campus, Kathmandu, 44600, Nepal
4 Statistics and Operations Research Department, College of Science, King Saud University, Riyadh, 11451, Kingdom of Saudi Arabia
5 Operations Research Department, Faculty of Graduate Studies for Statistical Research, Cairo University, Giza, 12613, Egypt
6 Wireless Intelligent Networks Center (WINC), School of Engineering and Applied Sciences, Nile University, Giza, 12588, Egypt
* Corresponding Author: Ali Wagdy Mohamed. Email:
(This article belongs to the Special Issue: Role of Computer in Modelling & Solving Real-World Problems)
Computers, Materials & Continua 2022, 70(3), 5467-5469. https://doi.org/10.32604/cmc.2022.020455
Received 25 May 2021; Accepted 01 August 2021; Issue published 11 October 2021
Abstract
COVID-19 is a growing problem worldwide with a high mortality rate. As a result, the World Health Organization (WHO) declared it a pandemic. In order to limit the spread of the disease, a fast and accurate diagnosis is required. A reverse transcript polymerase chain reaction (RT-PCR) test is often used to detect the disease. However, since this test is time-consuming, a chest computed tomography (CT) or plain chest X-ray (CXR) is sometimes indicated. The value of automated diagnosis is that it saves time and money by minimizing human effort. Three significant contributions are made by our research. Its initial purpose is to use the essential finetuning methodology to test the action and efficiency of a variety of vision models, ranging from Inception to Neural Architecture Search (NAS) networks. Second, by plotting class activation maps (CAMs) for individual networks and assessing classification efficiency with AUC-ROC curves, the behavior of these models is visually analyzed. Finally, stacked ensembles techniques were used to provide greater generalization by combining finetuned models with six ensemble neural networks. Using stacked ensembles, the generalization of the models improved. Furthermore, the ensemble model created by combining all of the finetuned networks obtained a state-of-the-art COVID-19 accuracy detection score of 99.17%. The precision and recall rates were 99.99% and 89.79%, respectively, highlighting the robustness of stacked ensembles. The proposed ensemble approach performed well in the classification of the COVID-19 lesions on CXR according to the experimental results.Keywords
Cite This Article
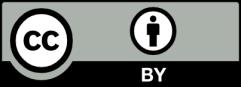