Open Access
ARTICLE
Swarm-Based Extreme Learning Machine Models for Global Optimization
1 Artificial Intelligence Department, Faculty of Computers and Artificial Intelligence, Benha University, Benha, 13518, Egypt
2 Scientific Computing Department, Faculty of Computers and Artificial Intelligence, Benha University, Benha, 13518, Egypt
* Corresponding Author: Mustafa Abdul Salam. Email:
(This article belongs to the Special Issue: Role of Machine Learning and Evolutionary Algorithms for Cancer Detection and Prediction)
Computers, Materials & Continua 2022, 70(3), 6339-6363. https://doi.org/10.32604/cmc.2022.020583
Received 30 May 2021; Accepted 29 July 2021; Issue published 11 October 2021
Abstract
Extreme Learning Machine (ELM) is popular in batch learning, sequential learning, and progressive learning, due to its speed, easy integration, and generalization ability. While, Traditional ELM cannot train massive data rapidly and efficiently due to its memory residence, high time and space complexity. In ELM, the hidden layer typically necessitates a huge number of nodes. Furthermore, there is no certainty that the arrangement of weights and biases within the hidden layer is optimal. To solve this problem, the traditional ELM has been hybridized with swarm intelligence optimization techniques. This paper displays five proposed hybrid Algorithms “Salp Swarm Algorithm (SSA-ELM), Grasshopper Algorithm (GOA-ELM), Grey Wolf Algorithm (GWO-ELM), Whale optimization Algorithm (WOA-ELM) and Moth Flame Optimization (MFO-ELM)”. These five optimizers are hybridized with standard ELM methodology for resolving the tumor type classification using gene expression data. The proposed models applied to the predication of electricity loading data, that describes the energy use of a single residence over a four-year period. In the hidden layer, Swarm algorithms are used to pick a smaller number of nodes to speed up the execution of ELM. The best weights and preferences were calculated by these algorithms for the hidden layer. Experimental results demonstrated that the proposed MFO-ELM achieved 98.13% accuracy and this is the highest model in accuracy in tumor type classification gene expression data. While in predication, the proposed GOA-ELM achieved 0.397which is least RMSE compared to the other models.Keywords
Cite This Article
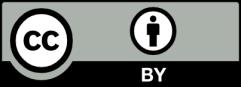