Open Access
ARTICLE
Optimized Stacked Autoencoder for IoT Enabled Financial Crisis Prediction Model
1 Department of Natural and Applied Sciences, College of Community-Aflaj, Prince Sattam bin Abdulaziz University, Saudi Arabia
2 Department of Information Systems, College of Computer and Information Sciences, Princess Nourah bint Abdulrahman University, Saudi Arabia
3 Department of Computer Science, King Khalid University, Muhayel Aseer, Saudi Arabia
4 Faculty of Computer and IT, Sana’a University, Sana’a, Yemen
5 Department of Computer and Self Development, Preparatory Year Deanship, Prince Sattam bin Abdulaziz University, AlKharj, Saudi Arabia
* Corresponding Author: Manar Ahmed Hamza. Email:
Computers, Materials & Continua 2022, 71(1), 1079-1094. https://doi.org/10.32604/cmc.2022.021199
Received 26 June 2021; Accepted 30 July 2021; Issue published 03 November 2021
Abstract
Recently, Financial Technology (FinTech) has received more attention among financial sectors and researchers to derive effective solutions for any financial institution or firm. Financial crisis prediction (FCP) is an essential topic in business sector that finds it useful to identify the financial condition of a financial institution. At the same time, the development of the internet of things (IoT) has altered the mode of human interaction with the physical world. The IoT can be combined with the FCP model to examine the financial data from the users and perform decision making process. This paper presents a novel multi-objective squirrel search optimization algorithm with stacked autoencoder (MOSSA-SAE) model for FCP in IoT environment. The MOSSA-SAE model encompasses different subprocesses namely pre-processing, class imbalance handling, parameter tuning, and classification. Primarily, the MOSSA-SAE model allows the IoT devices such as smartphones, laptops, etc., to collect the financial details of the users which are then transmitted to the cloud for further analysis. In addition, SMOTE technique is employed to handle class imbalance problems. The goal of MOSSA in SMOTE is to determine the oversampling rate and area of nearest neighbors of SMOTE. Besides, SAE model is utilized as a classification technique to determine the class label of the financial data. At the same time, the MOSSA is applied to appropriately select the ‘weights’ and ‘bias’ values of the SAE. An extensive experimental validation process is performed on the benchmark financial dataset and the results are examined under distinct aspects. The experimental values ensured the superior performance of the MOSSA-SAE model on the applied dataset.Keywords
Cite This Article
Citations
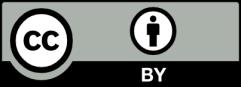