Open Access
ARTICLE
IoMT-Enabled Fusion-Based Model to Predict Posture for Smart Healthcare Systems
1 Faculty of Information Science and Technology, University Kebangsaan Malaysia, UKM, 43600, Selangor, Malaysia
2 Skyline University College, Sharjah, United Arab Emirates
* Corresponding Author: Taher M. Ghazal. Email:
Computers, Materials & Continua 2022, 71(2), 2579-2597. https://doi.org/10.32604/cmc.2022.019706
Received 22 April 2021; Accepted 26 August 2021; Issue published 07 December 2021
Abstract
Smart healthcare applications depend on data from wearable sensors (WSs) mounted on a patient’s body for frequent monitoring information. Healthcare systems depend on multi-level data for detecting illnesses and consequently delivering correct diagnostic measures. The collection of WS data and integration of that data for diagnostic purposes is a difficult task. This paper proposes an Errorless Data Fusion (EDF) approach to increase posture recognition accuracy. The research is based on a case study in a health organization. With the rise in smart healthcare systems, WS data fusion necessitates careful attention to provide sensitive analysis of the recognized illness. As a result, it is dependent on WS inputs and performs group analysis at a similar rate to improve diagnostic efficiency. Sensor breakdowns, the constant time factor, aggregation, and analysis results all cause errors, resulting in rejected or incorrect suggestions. This paper resolves this problem by using EDF, which is related to patient situational discovery through healthcare surveillance systems. Features of WS data are examined extensively using active and iterative learning to identify errors in specific postures. This technology improves position detection accuracy, analysis duration, and error rate, regardless of user movements. Wearable devices play a critical role in the management and treatment of patients. They can ensure that patients are provided with a unique treatment for their medical needs. This paper discusses the EDF technique for optimizing posture identification accuracy through multi-feature analysis. At first, the patients’ walking patterns are tracked at various time intervals. The characteristics are then evaluated in relation to the stored data using a random forest classifier.Keywords
Cite This Article
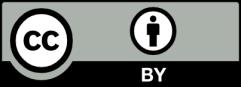