Open Access
ARTICLE
Evaluating the Efficiency of CBAM-Resnet Using Malaysian Sign Language
1 Faculty of Cognitive Sciences and Human Development, Universiti Malaysia Sarawak, Kuching, 94300, Malaysia
2 Hamdard Institute of Engineering and Technology, Islamabad, 44000, Pakistan
3 College of Computing and Information Technology, The University of Bisha, Bisha, 61922, Saudi Arabia
4 Department of Computer Sciences, Khushal Khan Khattak University, Karak, 27200, Pakistan
* Corresponding Author: Rehman Ullah Khan. Email:
(This article belongs to the Special Issue: Soft Computing and Machine Learning for Predictive Data Analytics)
Computers, Materials & Continua 2022, 71(2), 2755-2772. https://doi.org/10.32604/cmc.2022.022471
Received 09 August 2021; Accepted 27 September 2021; Issue published 07 December 2021
Abstract
The deaf-mutes population is constantly feeling helpless when others do not understand them and vice versa. To fill this gap, this study implements a CNN-based neural network, Convolutional Based Attention Module (CBAM), to recognise Malaysian Sign Language (MSL) in videos recognition. This study has created 2071 videos for 19 dynamic signs. Two different experiments were conducted for dynamic signs, using CBAM-3DResNet implementing ‘Within Blocks’ and ‘Before Classifier’ methods. Various metrics such as the accuracy, loss, precision, recall, F1-score, confusion matrix, and training time were recorded to evaluate the models’ efficiency. Results showed that CBAM-ResNet models had good performances in videos recognition tasks, with recognition rates of over 90% with little variations. CBAM-ResNet ‘Before Classifier’ is more efficient than ‘Within Blocks’ models of CBAM-ResNet. All experiment results indicated the CBAM-ResNet ‘Before Classifier’ efficiency in recognising Malaysian Sign Language and its worth of future research.Keywords
Cite This Article
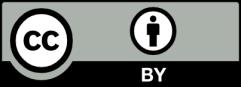