Open Access
ARTICLE
A BPR-CNN Based Hand Motion Classifier Using Electric Field Sensors
1 Department of Computer Science, Georgia State University, Atlanta, 30302, USA
2 Nat'l Program of Excellence in Software Centre, Chosun University, Gwangju, 61452, Korea
3 Department of Computer Inf. & Communication Eng., Chonnam Nat'l Univ., Gwangju, 61186, Korea
* Corresponding Author: Youngchul Kim. Email:
Computers, Materials & Continua 2022, 71(3), 5413-5425. https://doi.org/10.32604/cmc.2022.023172
Received 30 August 2021; Accepted 18 November 2021; Issue published 14 January 2022
Abstract
In this paper, we propose a BPR-CNN (Biometric Pattern Recognition-Convolution Neural Network) classifier for hand motion classification as well as a dynamic threshold algorithm for motion signal detection and extraction by EF (Electric Field) sensors. Currently, an EF sensor or EPS (Electric Potential Sensor) system is attracting attention as a next-generation motion sensing technology due to low computation and price, high sensitivity and recognition speed compared to other sensor systems. However, it remains as a challenging problem to accurately detect and locate the authentic motion signal frame automatically in real-time when sensing body-motions such as hand motion, due to the variance of the electric-charge state by heterogeneous surroundings and operational conditions. This hinders the further utilization of the EF sensing; thus, it is critical to design the robust and credible methodology for detecting and extracting signals derived from the motion movement in order to make use and apply the EF sensor technology to electric consumer products such as mobile devices. In this study, we propose a motion detection algorithm using a dynamic offset-threshold method to overcome uncertainty in the initial electrostatic charge state of the sensor affected by a user and the surrounding environment of the subject. This method is designed to detect hand motions and extract its genuine motion signal frame successfully with high accuracy. After setting motion frames, we normalize the signals and then apply them to our proposed BPR-CNN motion classifier to recognize their motion types. Conducted experiment and analysis show that our proposed dynamic threshold method combined with a BPR-CNN classifier can detect the hand motions and extract the actual frames effectively with 97.1% accuracy, 99.25% detection rate, 98.4% motion frame matching rate and 97.7% detection & extraction success rate.Keywords
Cite This Article
Citations
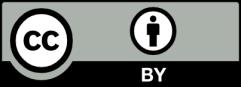