Open Access
ARTICLE
An Efficient Intrusion Detection Framework in Software-Defined Networking for Cybersecurity Applications
1 Department of Computer Science, Community College, King Saud University, Riyadh, 28095, Saudi Arabia
2 Deanship of Scientific Research, Saudi Electronic University, Riyadh, Saudi Arabia
3 Management Information System Department, College of Business Administration, King Saud University, Riyadh, 28095, Saudi Arabia
4 Department of Computer Science and Engineering, Faculty of Electronic Engineering, Menoufia University, Menouf, 32952, Egypt
5 Security Engineering Lab, Computer Science Department, Prince Sultan University, Riyadh, 11586, Saudi Arabia
6 Electronics and Electrical Communications Eng. Depart., Faculty of Electronic Engineering, Menoufia University, Menouf, 32952, Egypt
* Corresponding Author: Walid El-Shafai. Email:
Computers, Materials & Continua 2022, 72(2), 3529-3548. https://doi.org/10.32604/cmc.2022.025262
Received 18 November 2021; Accepted 24 January 2022; Issue published 29 March 2022
Abstract
Network management and multimedia data mining techniques have a great interest in analyzing and improving the network traffic process. In recent times, the most complex task in Software Defined Network (SDN) is security, which is based on a centralized, programmable controller. Therefore, monitoring network traffic is significant for identifying and revealing intrusion abnormalities in the SDN environment. Consequently, this paper provides an extensive analysis and investigation of the NSL-KDD dataset using five different clustering algorithms: K-means, Farthest First, Canopy, Density-based algorithm, and Exception-maximization (EM), using the Waikato Environment for Knowledge Analysis (WEKA) software to compare extensively between these five algorithms. Furthermore, this paper presents an SDN-based intrusion detection system using a deep learning (DL) model with the KDD (Knowledge Discovery in Databases) dataset. First, the utilized dataset is clustered into normal and four major attack categories via the clustering process. Then, a deep learning method is projected for building an efficient SDN-based intrusion detection system. The results provide a comprehensive analysis and a flawless reasonable study of different kinds of attacks incorporated in the KDD dataset. Similarly, the outcomes reveal that the proposed deep learning method provides efficient intrusion detection performance compared to existing techniques. For example, the proposed method achieves a detection accuracy of 94.21% for the examined dataset.Keywords
Cite This Article
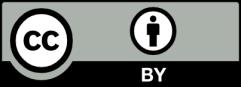