Open Access
ARTICLE
Brain Tumor Auto-Segmentation on Multimodal Imaging Modalities Using Deep Neural Network
1,2 Department of Software Engineering, Daffodil International University, Dhaka, 1207, Bangladesh
3 Department of Computing and Information System, Daffodil International University, Dhaka, 1207, Bangladesh
4 Department of Computer Science & Engineering, BRAC University, Dhaka, 1212, Bangladesh
5 Department of Information and Communication Engineering, Bangladesh University of Professionals (BUP), Dhaka,
1216, Bangladesh
6 Department of Computer Engineering, College of Computers and Information Technology, Taif University, P.O. Box
11099, Taif 21944, Saudi Arabia
7 Department of Information Technology, College of Computers and Information Technology, Taif University, P.O. Box
11099, Taif 21944, Saudi Arabia
8 Department of Electrical Engineering, College of Engineering, Taif University, P.O. Box 11099, Taif 21944, Saudi Arabia
9 Electronics and Electrical Communications Engineering Department, Faculty of Electronic Engineering, Menouf, 32951,
Egypt
* Corresponding Author: Ahmed Nabih Zaki Rashed. Email:
Computers, Materials & Continua 2022, 72(3), 4509-4523. https://doi.org/10.32604/cmc.2022.025977
Received 11 December 2021; Accepted 16 February 2022; Issue published 21 April 2022
Abstract
Due to the difficulties of brain tumor segmentation, this paper proposes a strategy for extracting brain tumors from three-dimensional Magnetic Resonance Image (MRI) and Computed Tomography (CT) scans utilizing 3D U-Net Design and ResNet50, taken after by conventional classification strategies. In this inquire, the ResNet50 picked up accuracy with 98.96%, and the 3D U-Net scored 97.99% among the different methods of deep learning. It is to be mentioned that traditional Convolutional Neural Network (CNN) gives 97.90% accuracy on top of the 3D MRI. In expansion, the image fusion approach combines the multimodal images and makes a fused image to extricate more highlights from the medical images. Other than that, we have identified the loss function by utilizing several dice measurements approach and received Dice Result on top of a specific test case. The average mean score of dice coefficient and soft dice loss for three test cases was 0.0980. At the same time, for two test cases, the sensitivity and specification were recorded to be 0.0211 and 0.5867 using patch level predictions. On the other hand, a software integration pipeline was integrated to deploy the concentrated model into the webserver for accessing it from the software system using the Representational state transfer (REST) API. Eventually, the suggested models were validated through the Area Under the Curve–Receiver Characteristic Operator (AUC–ROC) curve and Confusion Matrix and compared with the existing research articles to understand the underlying problem. Through Comparative Analysis, we have extracted meaningful insights regarding brain tumour segmentation and figured out potential gaps. Nevertheless, the proposed model can be adjustable in daily life and the healthcare domain to identify the infected regions and cancer of the brain through various imaging modalities.Keywords
Cite This Article
E. Hossain, M. Shazzad Hossain, M. Selim Hossain, S. Al Jannat, M. Huda et al., "Brain tumor auto-segmentation on multimodal imaging modalities using deep neural network," Computers, Materials & Continua, vol. 72, no.3, pp. 4509–4523, 2022. https://doi.org/10.32604/cmc.2022.025977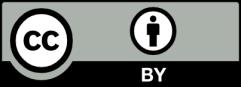