Open Access
ARTICLE
Hybrid Single Image Super-Resolution Algorithm for Medical Images
1 Department of Electronics and Electrical Communications Engineering, Faculty of Electronic Engineering, Menoufia University, Menouf, 32952, Egypt
2 Security Engineering Lab, Computer Science Department, Prince Sultan University, Riyadh, 11586, Saudi Arabia
3 Electrical Engineering Department, College of Engineering, Prince Sattam Bin Abdulaziz University, Wadi Addwasir, 11991, Saudi Arabia
4 Electrical Engineering Department, Aswan University, Aswan, 81542, Egypt
5 Electronics and Micro-Electronics Laboratory (E. μ. E. L), Faculty of Sciences, University of Monastir, Monastir, 5000, Tunisia
6 Alexandria Higher Institute of Engineering & Technology (AIET), Alexandria, Egypt
7 Electronics and Communications Engineering Department, College of Engineering and Technology, Arab Academy for Science, Technology and Maritime Transport, Alexandria, 1029, Egypt
* Corresponding Author: Ehab Mahmoud Mohamed. Email:
Computers, Materials & Continua 2022, 72(3), 4879-4896. https://doi.org/10.32604/cmc.2022.028364
Received 08 February 2022; Accepted 14 March 2022; Issue published 21 April 2022
Abstract
High-quality medical microscopic images used for diseases detection are expensive and difficult to store. Therefore, low-resolution images are favorable due to their low storage space and ease of sharing, where the images can be enlarged when needed using Super-Resolution (SR) techniques. However, it is important to maintain the shape and size of the medical images while enlarging them. One of the problems facing SR is that the performance of medical image diagnosis is very poor due to the deterioration of the reconstructed image resolution. Consequently, this paper suggests a multi-SR and classification framework based on Generative Adversarial Network (GAN) to generate high-resolution images with higher quality and finer details to reduce blurring. The proposed framework comprises five GAN models: Enhanced SR Generative Adversarial Networks (ESRGAN), Enhanced deep SR GAN (EDSRGAN), Sub-Pixel-GAN, SRGAN, and Efficient Wider Activation-B GAN (WDSR-b-GAN). To train the proposed models, we have employed images from the famous BreakHis dataset and enlarged them by 4× and 16× upscale factors with the ground truth of the size of 256 × 256 × 3. Moreover, several evaluation metrics like Peak Signal-to-Noise Ratio (PSNR), Mean Squared Error (MSE), Structural Similarity Index (SSIM), Multiscale Structural Similarity Index (MS-SSIM), and histogram are applied to make comprehensive and objective comparisons to determine the best methods in terms of efficiency, training time, and storage space. The obtained results reveal the superiority of the proposed models over traditional and benchmark models in terms of color and texture restoration and detection by achieving an accuracy of 99.7433%.Keywords
Cite This Article
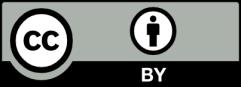