Open Access
ARTICLE
Diabetes Prediction Using Derived Features and Ensembling of Boosting Classifiers
1 School of Computing, SRM Institute of Science and Technology, Kattankulathur, Chennai, India
2 School of Computing, Bharat Institute of Higher Education and Research, Chennai, India
3 School of Computing, University of Eastern Finland, Kuopio, Finland
* Corresponding Author: R. Rajkamal. Email:
Computers, Materials & Continua 2022, 73(1), 2013-2033. https://doi.org/10.32604/cmc.2022.027142
Received 11 January 2022; Accepted 04 March 2022; Issue published 18 May 2022
Abstract
Diabetes is increasing commonly in people’s daily life and represents an extraordinary threat to human well-being. Machine Learning (ML) in the healthcare industry has recently made headlines. Several ML models are developed around different datasets for diabetic prediction. It is essential for ML models to predict diabetes accurately. Highly informative features of the dataset are vital to determine the capability factors of the model in the prediction of diabetes. Feature engineering (FE) is the way of taking forward in yielding highly informative features. Pima Indian Diabetes Dataset (PIDD) is used in this work, and the impact of informative features in ML models is experimented with and analyzed for the prediction of diabetes. Missing values (MV) and the effect of the imputation process in the data distribution of each feature are analyzed. Permutation importance and partial dependence are carried out extensively and the results revealed that Glucose (GLUC), Body Mass Index (BMI), and Insulin (INS) are highly informative features. Derived features are obtained for BMI and INS to add more information with its raw form. The ensemble classifier with an ensemble of AdaBoost (AB) and XGBoost (XB) is considered for the impact analysis of the proposed FE approach. The ensemble model performs well for the inclusion of derived features provided the high Diagnostics Odds Ratio (DOR) of 117.694. This shows a high margin of 8.2% when compared with the ensemble model with no derived features (DOR = 96.306) included in the experiment. The inclusion of derived features with the FE approach of the current state-of-the-art made the ensemble model performs well with Sensitivity (0.793), Specificity (0.945), DOR (79.517), and False Omission Rate (0.090) which further improves the state-of-the-art results.Keywords
Cite This Article
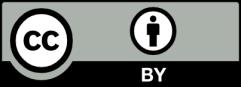