Open Access
ARTICLE
An Effective Memory Analysis for Malware Detection and Classification
Department of Information Science & Technology, Universiti Kebangsaan Malaysia, Selangor, 43600, Malaysia
* Corresponding Author: Rami Sihwail. Email:
Computers, Materials & Continua 2021, 67(2), 2301-2320. https://doi.org/10.32604/cmc.2021.014510
Received 25 September 2020; Accepted 08 November 2020; Issue published 05 February 2021
Abstract
The study of malware behaviors, over the last years, has received tremendous attention from researchers for the purpose of reducing malware risks. Most of the investigating experiments are performed using either static analysis or behavior analysis. However, recent studies have shown that both analyses are vulnerable to modern malware files that use several techniques to avoid analysis and detection. Therefore, extracted features could be meaningless and a distraction for malware analysts. However, the volatile memory can expose useful information about malware behaviors and characteristics. In addition, memory analysis is capable of detecting unconventional malware, such as in-memory and fileless malware. However, memory features have not been fully utilized yet. Therefore, this work aims to present a new malware detection and classification approach that extracts memory-based features from memory images using memory forensic techniques. The extracted features can expose the malware’s real behaviors, such as interacting with the operating system, DLL and process injection, communicating with command and control site, and requesting higher privileges to perform specific tasks. We also applied feature engineering and converted the features to binary vectors before training and testing the classifiers. The experiments show that the proposed approach has a high classification accuracy rate of 98.5% and a false positive rate as low as 1.24% using the SVM classifier. The efficiency of the approach has been evaluated by comparing it with other related works. Also, a new memory-based dataset consisting of 2502 malware files and 966 benign samples forming 8898 features and belonging to six memory types has been created and published online for research purposes.Keywords
Cite This Article
Citations
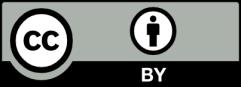