Open Access
ARTICLE
Air Pollution Prediction Via Graph Attention Network and Gated Recurrent Unit
1 Beijing Key Laboratory of Multimedia and Intelligent Software Technology, Beijing Artificial Intelligence Institute, the Faculty of Information Technology, Beijing University of Technology, Beijing, 100124, China
2 Beijing Meteorological Observatory, Beijing, 100089, China
3 Nanjing University of Information Science & Technology, Nanjing, 210044, China
4 China Meteorological Administration Training Centre, Beijing, 100081, China
5 Texas Tech University, Lubbock, TX79409, United States
* Corresponding Author: Yong Zhang. Email:
Computers, Materials & Continua 2022, 73(1), 673-687. https://doi.org/10.32604/cmc.2022.028411
Received 09 February 2022; Accepted 23 March 2022; Issue published 18 May 2022
Abstract
PM2.5 concentration prediction is of great significance to environmental protection and human health. Achieving accurate prediction of PM2.5 concentration has become an important research task. However, PM2.5 pollutants can spread in the earth’s atmosphere, causing mutual influence between different cities. To effectively capture the air pollution relationship between cities, this paper proposes a novel spatiotemporal model combining graph attention neural network (GAT) and gated recurrent unit (GRU), named GAT-GRU for PM2.5 concentration prediction. Specifically, GAT is used to learn the spatial dependence of PM2.5 concentration data in different cities, and GRU is to extract the temporal dependence of the long-term data series. The proposed model integrates the learned spatio-temporal dependencies to capture long-term complex spatio-temporal features. Considering that air pollution is related to the meteorological conditions of the city, the knowledge acquired from meteorological data is used in the model to enhance PM2.5 prediction performance. The input of the GAT-GRU model consists of PM2.5 concentration data and meteorological data. In order to verify the effectiveness of the proposed GAT-GRU prediction model, this paper designs experiments on real-world datasets compared with other baselines. Experimental results prove that our model achieves excellent performance in PM2.5 concentration prediction.Keywords
Cite This Article
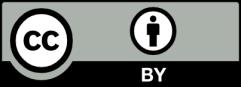