Open Access
ARTICLE
HDLIDP: A Hybrid Deep Learning Intrusion Detection and Prevention Framework
1 Computer Engineering and Systems Department, Faculty of Engineering, Mansoura University, Mansoura, 35516, DK, Egypt
2 Head of Communications and Computer Engineering Department, MISR Higher Institute for Engineering and Technology, Mansoura, 35111, DK, Egypt
3 Mechatronics Department, Faculty of Engineering, Horus University in Egypt (HUE), New Damietta, 34517, DT, Egypt
* Corresponding Author: Magdy M. Fadel. Email:
Computers, Materials & Continua 2022, 73(2), 2293-2312. https://doi.org/10.32604/cmc.2022.028287
Received 07 February 2022; Accepted 25 March 2022; Issue published 16 June 2022
Abstract
Distributed denial-of-service (DDoS) attacks are designed to interrupt network services such as email servers and webpages in traditional computer networks. Furthermore, the enormous number of connected devices makes it difficult to operate such a network effectively. Software defined networks (SDN) are networks that are managed through a centralized control system, according to researchers. This controller is the brain of any SDN, composing the forwarding table of all data plane network switches. Despite the advantages of SDN controllers, DDoS attacks are easier to perpetrate than on traditional networks. Because the controller is a single point of failure, if it fails, the entire network will fail. This paper offers a Hybrid Deep Learning Intrusion Detection and Prevention (HDLIDP) framework, which blends signature-based and deep learning neural networks to detect and prevent intrusions. This framework improves detection accuracy while addressing all of the aforementioned problems. To validate the framework, experiments are done on both traditional and SDN datasets; the findings demonstrate a significant improvement in classification accuracy.Keywords
Cite This Article
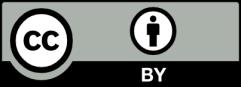