Open Access
ARTICLE
Enhancing PDF Malware Detection through Logistic Model Trees
Department of Information Systems, King Abdulaziz University, P.O. Box 80217, Jeddah, 21589, Saudi Arabia
* Corresponding Author: Muhammad Binsawad. Email:
(This article belongs to the Special Issue: AI and Data Security for the Industrial Internet)
Computers, Materials & Continua 2024, 78(3), 3645-3663. https://doi.org/10.32604/cmc.2024.048183
Received 30 November 2023; Accepted 22 January 2024; Issue published 26 March 2024
Abstract
Malware is an ever-present and dynamic threat to networks and computer systems in cybersecurity, and because of its complexity and evasiveness, it is challenging to identify using traditional signature-based detection approaches. The study article discusses the growing danger to cybersecurity that malware hidden in PDF files poses, highlighting the shortcomings of conventional detection techniques and the difficulties presented by adversarial methodologies. The article presents a new method that improves PDF virus detection by using document analysis and a Logistic Model Tree. Using a dataset from the Canadian Institute for Cybersecurity, a comparative analysis is carried out with well-known machine learning models, such as Credal Decision Tree, Naïve Bayes, Average One Dependency Estimator, Locally Weighted Learning, and Stochastic Gradient Descent. Beyond traditional structural and JavaScript-centric PDF analysis, the research makes a substantial contribution to the area by boosting precision and resilience in malware detection. The use of Logistic Model Tree, a thorough feature selection approach, and increased focus on PDF file attributes all contribute to the efficiency of PDF virus detection. The paper emphasizes Logistic Model Tree’s critical role in tackling increasing cybersecurity threats and proposes a viable answer to practical issues in the sector. The results reveal that the Logistic Model Tree is superior, with improved accuracy of 97.46% when compared to benchmark models, demonstrating its usefulness in addressing the ever-changing threat landscape.Keywords
Cite This Article
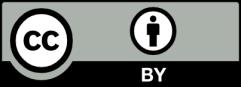