Open Access
ARTICLE
Transfer Learning for Chest X-rays Diagnosis Using Dipper Throated Algorithm
1 Department of Computer Sciences, College of Computer and Information Sciences, Princess Nourah Bint Abdulrahman University, Riyadh, 11671, Saudi Arabia
2 Department of Communications and Electronics, Delta Higher Institute of Engineering and Technology, Mansoura, 35111, Egypt
3 Faculty of Artificial Intelligence, Delta University for Science and Technology, Mansoura, 35712, Egypt
4 Electronics and Communications Engineering Department, Faculty of Engineering, Delta University for Science and Technology, Mansoura, 35712, Egypt
5 Department of Computer Science, Faculty of Computer and Information Sciences, Ain Shams University, Cairo, 11566, Egypt
6 Department of Computer Science, College of Computing and Information Technology, Shaqra University, 11961, Saudi Arabia
* Corresponding Author: Amel Ali Alhussan. Email:
Computers, Materials & Continua 2022, 73(2), 2371-2387. https://doi.org/10.32604/cmc.2022.030447
Received 26 March 2022; Accepted 26 April 2022; Issue published 16 June 2022
Abstract
Most children and elderly people worldwide die from pneumonia, which is a contagious illness that causes lung ulcers. For diagnosing pneumonia from chest X-ray images, many deep learning models have been put forth. The goal of this research is to develop an effective and strong approach for detecting and categorizing pneumonia cases. By varying the deep learning approach, three pre-trained models, GoogLeNet, ResNet18, and DenseNet121, are employed in this research to extract the main features of pneumonia and normal cases. In addition, the binary dipper throated optimization (DTO) algorithm is utilized to select the most significant features, which are then fed to the K-nearest neighbor (KNN) classifier for getting the final classification decision. To guarantee the best performance of KNN, its main parameter (K) is optimized using the continuous DTO algorithm. To test the proposed approach, six evaluation metrics were employed namely, positive and negative predictive values, accuracy, specificity, sensitivity, and F1-score. Moreover, the proposed approach is compared with other traditional approaches, and the findings confirmed the superiority of the proposed approach in terms of all the evaluation metrics. The minimum accuracy achieved by the proposed approach is (98.5%), and the maximum accuracy is (99.8%) when different test cases are included in the evaluation experiments.Keywords
Cite This Article
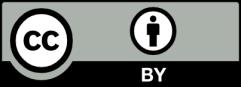