Open Access
ARTICLE
Transfer Learning for Disease Diagnosis from Myocardial Perfusion SPECT Imaging
1 Institute of Information Technology, AMST, Hanoi, 11307, Vietnam
2 Department of Medical Equipment, 108 Military Central Hospital, Hanoi, 11610, Vietnam
* Corresponding Author: Nguyen Chi Thanh. Email:
Computers, Materials & Continua 2022, 73(3), 5925-5941. https://doi.org/10.32604/cmc.2022.031027
Received 08 April 2022; Accepted 07 June 2022; Issue published 28 July 2022
Abstract
Coronary artery disease (CAD) is one of the most common pathological conditions and the major global cause of death. Myocardial perfusion imaging (MPI) using single-photon emission computed tomography (SPECT) is a non-invasive method and plays an essential role in diagnosing CAD. However, there is currently a shortage of doctors who can diagnose using SPECT-MPI in developing countries, especially Vietnam. Research on deploying machine learning and deep learning in supporting CAD diagnosis has been noticed for a long time. However, these methods require a large dataset and are therefore time-consuming and labor-intensive. This study aims to develop a cost-effective and high-performance CAD classification model to support doctors in these countries. In this paper, we propose a transfer learning framework for a multi-stage training process with different learning rates. The process consists of two training stages: a warming up stage in which all layers of a pre-trained model (on ImageNet dataset) are frozen; and a fine-tuning stage in which a small amount of the top layers are unfrozen and then retrained with a lower learning rate. The dataset for this study consists of the polar maps from 218 patients. Various popular CNN-based pre-trained models have been investigated, and ResNet152V2-based model has obtained the highest performances with an accuracy of 95.5%, area under the receiver operating characteristic curve (AUC) score 0.932, sensitivity 94.4%, precision 96.4%, and F1-score 95.2%. These performances are competitive or even better than all state-of-the-art approaches in terms of classification accuracy and sensitivity. We also apply the class activation mapping technique to help explain the model’s predictions and increase the model’s reliability, proving capable of assisting the SPECT image readers in the CAD diagnosis.Keywords
Cite This Article
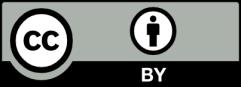