Open Access
ARTICLE
Towards Fully Secure 5G Ultra-Low Latency Communications: A Cost-Security Functions Analysis
1 Universidad Politécnica de Madrid, Madrid, España
2 Argonne National Laboratory, Lemont, IL, USA
3 Universidad de Panamá, Panamá, Panamá
* Corresponding Author: Borja Bordel. Email:
Computers, Materials & Continua 2023, 74(1), 855-880. https://doi.org/10.32604/cmc.2023.026787
Received 05 January 2022; Accepted 06 June 2022; Issue published 22 September 2022
Abstract
Future components to enhance the basic, native security of 5G networks are either complex mechanisms whose impact in the requiring 5G communications are not considered, or lightweight solutions adapted to ultra-reliable low-latency communications (URLLC) but whose security properties remain under discussion. Although different 5G network slices may have different requirements, in general, both visions seem to fall short at provisioning secure URLLC in the future. In this work we address this challenge, by introducing cost-security functions as a method to evaluate the performance and adequacy of most developed and employed non-native enhanced security mechanisms in 5G networks. We categorize those new security components into different groups according to their purpose and deployment scope. We propose to analyze them in the context of existing 5G architectures using two different approaches. First, using model checking techniques, we will evaluate the probability of an attacker to be successful against each security solution. Second, using analytical models, we will analyze the impact of these security mechanisms in terms of delay, throughput consumption, and reliability. Finally, we will combine both approaches using stochastic cost-security functions and the PRISM model checker to create a global picture. Our results are first evidence of how a 5G network that covers and strengthened all security areas through enhanced, dedicated non-native mechanisms could only guarantee secure URLLC with a probability of ∼55%.Keywords
5G communication networks [1] are envisioned to provide innovative services to a large catalogue of scenarios ranging from traditional end-user communications (phone calls, web navigation, etc.) to Industry 4.0 [2] and Cyber-Physical Systems [3] and, even, critical infrastructures and applications. In general, to be considered a 5G network, a communication service provider must support three main services regarding the Quality-of-Service:
• Enhanced Mobile Broadband Communications (eMBBC) [4]: Applications and users must be provided with stable bit rates above 100 Mbps, being also possible peaks around 10 Gbps.
• Ultra-reliable Low-Latency Communications (URLLC) [5]: 5G networks must present an end-to-end delay below five milliseconds.
• Massive machine-type communications (mMTC) [6]: Some envisioned applications may present highly dense scenarios with up to one million devices per squared kilometer. 5G services must be able to operate in these conditions.
These requirements are mandatory for all network configurations and implementations (standalone or non-standalone). Briefly, in the transition from 4G to 5G networks, all network segments and components are aimed to move towards service-based open architectures, including security services, being able to cooperate and interoperate together. This change affects core networks in a more relevant way, as more changes are needed from monolithic closed 4G cores. Regarding Radio Access Networks (RAN), some 4G implementations have already achieved certain decentralization and distribution level, but 5G solutions must still improve the current openness of 4G RAN.
Although in previous mobile networks (mainly 3G and 4G) security solutions cover a wide catalogue of aspects and potential attacks, their main focus are authentication and integrity solutions. This analysis is still critical and valid in future 5G networks [7], but new factors are added and must be considered: 1) very strict Quality-of-Service (QoS) requirements must be guaranteed and 2) 5G services (URLLC, eMBBC, and mMTC) consume most resources in the network. At the same time, the entire world is moving towards a scenario where mobile communications are the main communication service and technologies. Furthermore, these future mobile technologies are being integrated into critical applications, so they must face new and critical attacks [8], while other threats such as cyberterrorism do not decline but get stronger. This context pushes designers, service providers and users to strengthen security in 5G solutions [8] through non-native but essential components. In this context, many researchers and industry leaders have initiated the design of a completely new ecosystem of security services and non-native components for 5G networks. However, most of these solutions face a critical problem. Strongest security mechanisms tend to be computationally heavy and quite slow, turning more difficult, or even impossible, to meet the expected QoS requirements in 5G networks, given physical technologies cannot provide more capacity or improve their performance. On the other hand, lightweight solutions (whose impact is reduced and could be, eventually, compensated with some network optimization policies) are questionable because of their practical weaknesses against statistical and reverse engineering attacks [9]. In traditional mobile network where computational resources are abundant, complex technologies are easily deployed. However, in future 5G networks some network slices incorporate dense deployments of resource-constrained devices, while other slices are highly demanding in terms of strong QoS requirements (i.e., resources will not be as abundant as now). In this future close-fitting context, any unexpected behavior (from the mobile network, but especially from those non-native security components) would make unfeasible to provide eMBBC and, mainly, URLLC [10].
Given the currently proposed 5G open architectures and physical technologies (very close-fitting, even for 4G+ services and QoS requirements) and the current state of non-native components to provide enhanced protection to 5G services and users (either very heavy and complex, or not fully secure), we hypothesize that it will be unfeasible to provide fully secure URLLC services.
An initial step to address this problem is to define a joint evaluation framework, in which the advantages (protections level) and disadvantages (impact on QoS) of security solutions for 5G networks are analyzed in the proper context, i.e., the future network architectures. Using this combined analysis, we can define boundaries to the opportunity-cost balance and clearly show which proposed security solutions are valid, which design principles are adequate, and which solutions must be discarded either because of their poor security performance or because of their unaffordable computational cost. Therefore, in this paper we analyze the performance of proposed security mechanisms for 5G networks. We propose a stochastic cost-security function, which combines both the reached protection level and the impact in the provided QoS.
We analyzed the impact security mechanisms have on service quality through relevant key performance indicators (KPI), defined in a probabilistic manner (with a special focus on those parameters that affect the key features of 5G services such as URLLC). We deducted this impact numerically, using analytical models that describe the behavior of relevant variables such as the end-to-end delay and jitter. These models are built from basic general communication schemes, that are increasingly enriched when we analyze the internal structure of future 5G networks and the characteristics of future application scenarios. We define and study different models, considering the most common and promising 5G implementations, and the most relevant scientific proposals [11]. We analyzed proposed security solutions according to their scope and purpose, classifying reported security mechanisms in nine different groups. For each group, we identified and modeled the most relevant attacks and their corresponding adversary models. In the context of the aforementioned architectures, we will analyze these security components and adversary models using logic rules and probabilistic model checkers. As a result, we developed a catalogue of probabilistic functions for the most important security components in future 5G networks. Probabilistic functions are then combined in a cost-security stochastic function, that represents the enabled QoS (as a probability distribution function) for a given security configuration. In order to make decisions, different significance levels may be considered, representing the different QoS and/or critical character of the different applications under study.
The contributions of this paper are the following:
1. Analytical models for most developed open, service-based 5G architectures (in terms of reported experiments and experiences with those architectures), including core and RAN solutions.
2. Analytical models for most developed non-native protection components for 5G networks and enhanced secure communications.
3. A cost-security function that combines both 5G architecture and security solution models to determine the feasibility of URLLC service provisioning in the presence of security mechanism.
4. A numerical analysis using the PRISM model checker that shows in general, a 5G network deploying all security mechanisms can only guarantee URLLC with a probability of ∼55%.
The proposed models are based on open service-architectures available by the writing of this paper. Future versions and 5G specification may require additional adjustments to the models. The global and final goal of this analytical model is to provide a systematic and quantitative approach to evaluate the feasibility of fully secure 5G URLLC, considering different network architectures and the most relevant reported non-native security components nowadays. Through this model, any potential stakeholder may evaluate and compare their own security architecture with the state of the art using quantitative metrics.
The provided analysis aims to answer three basic research questions:
• REQ#1: Can secure URLLC be guaranteed by the best existing technologies for 5G networks?
• REQ#2: What is the success probability of secure URLLC in 5G core networks in the current technological state?
• REQ#3: What is the success probability of secure URLLC if 5G endpoints implement current security mechanisms?
The rest of the paper is organized as follows: Section 2 describes the state of the art on 5G security analysis and benchmarking. Section 3 describes the main study, from the 5G architectural description and model creation to the analysis and modeling of different security mechanisms for 5G networks and their evaluation through model checking techniques. Section 4 shows numerical results for the selected scenarios. Finally, Section 5 presents our conclusions.
2 State of the Art on 5G and 4G Security Analysis Frameworks
Although different studies have already addressed the key security-relevant aspects of future and current 5G deployments [12], only few studies, by the writing of this paper, have analyzed in a scientific manner the performance of 5G non-native security components (despite the fact they are considered essential in most application scenarios [13]). Besides, sparse works have studied the balance between security and QoS, in order to evaluate 5G solutions in real situations and clarify the future of secure URLLC. Initial proposals are focused on improving reliability and security in the radio segment in 5G networks [11] from a theoretical point of view and disregarding other important aspect of QoS (e.g., latency).
2.1 5G Security Solutions and Analysis Frameworks
In general, works on 5G networks and secure URLLC are exploratory and qualitative. Ahmad et al. [14] described the threats and challenges future 5G security mechanism must address, while Yoshizawa et al. [15] exposed the difficulty of providing security services compatible with URLLC. Some articles discussing, from a qualitative point of view, how different solutions at different levels may improve the QoS of secure URLLC in 5G networks may be also found. Different proposals belong to this group: physical layer architectures [16], resource allocation algorithms [17], intelligent 5G-oriented technologies [18], among others. Despite these previous works, only few studies analyze from a quantitative and scientific point of view the impact of security mechanisms on URLLC. However, their general conclusion is that virtualization-based networks cannot easily support URLLC, and bare metal schemes must be preferred [19]. This approach, nevertheless, evolves contrary to market tendencies (more focused on software-defined networks). Besides, not all hardware infrastructures have proven to be adequate, and additional requirements such as special operating systems [20] and CPU features [21] have been identified. Once more, this view is the opposite to the use of generic devices that the current technological market envisions. Moreover, URLLC has an intrinsic probabilistic definition that most studies ignore. They typically evaluate their solutions against traditional conditions such as network congestion [22], while often ignoring network reliability.
A different approach is to consider lightweight solutions that, potentially, may be compatible with URLLC [9]. Despite achievable latencies and error rates are very low, these solutions have not been integrated in a real 5G architecture to clarify whether they are compatible with 5G requirements. On the contrary, most of these schemes have shown important security weaknesses [23].
2.2 4G Security Solutions and Analysis Frameworks
On the other hand, although this paper is focused on 5G scenarios, security models are also applicable to previous mobile generations such as 4G LTE (Long-Term Evolution). Specifically, different models for native security protocols in 4G mobile networks have been proposed [24], including analyses from different perspectives and at different levels. In that way, we can find explicit numerical models [24], parametric models that are trained for specific situations [25] or statistical frameworks [26], all of them modeling the behavior of security protocols such as Radio Resource Control (RRC). This approach, although very relevant as it can be used to prevent cyberattacks and network weaknesses, has a little impact on a URLLC feasibility analysis, as native protocols are already optimized and designed to allow 5G URLLC. Furthermore, the most common models regarding security aspects and LTE are attacker models [27]. In this works, the security models describe the behaver of an eventual attacker, not the network operations. This strategy has no impact on feasibility analyses.
In this paper we address this gap in the existing literature by proposing a new methodology based on cost-security functions to study the feasibility of URLLC in fully secure 5G networks (including, specifically, non-native security components).
In this section, we propose and describe the analytical models, functions, and methodology of this study. Section 3.1 describes the most relevant 5G core and RAN implementations and, for each one, it proposes an analytic model describing its operation. Section 3.2 presents the current security solutions reported for future 5G networks and the most relevant related attacks. Section 3.3 describes the formal security analysis methodology, based on model checking. Finally, Section 3.4 proposes the global cost-security functions to evaluate the viability of secure URLLC.
3.1 5G Core and RAN Implementations: Analytical Models
In order to evaluate the feasibility of URLLC in 5G networks in the presence of non-native security components (what we have called, fully secure URLLC), we must consider components in the context of a particular network architecture. In fact, the internal structure of networks and the required interactions among network modules highly condition the global performance (delay, jitter, etc.) and then, the feasibility of URLLC.
Modern mobile networks, including future 5G solutions, are separated into two different network domains: the radio access network (RAN), focused on providing local wireless connectivity to mobile users and the core network, managing global connectivity. The 3GPP Release 15 [28] standard provides specifications for the next-generation radio access network (NG-RAN) known as New Radio (NR) and a next generation core network known as 5G Core (5GC). Thus, in order to simplify the model definition process, we first analyze each network domain separately. Parameters with super index “core” are associated to the 5G core network, while parameters with super index RAN are related to the radio access network.
In this work we consider the three basic indicators that characterize URLLC: end-to-end delay (latency) D, jitter J, and reliability R (understood as the probability of a data packet to be successfully transmitted and delivered). Global indicators, then, may be easily obtained from measurements associated to core network and RAN through the probability theory (1).
Parameters
The 5G Core network is expected to be an IP backbone over optical transport. Two types of delays manifest in core network links: the transmission delay
Given a packet
Furthermore, the transmitter and the receiver in a link, may manage packets from different services and users, so the packet
To calculate the delays associated to queues we use a Poisson model M/M/1/N (in Kendall notation), assuming that packet generation
The propagation delay in an optical fiber link depends on the length of the link d and the physical characteristics of the material, represented by the delay per kilometer ratio
Previous delays are totally dependent on the module
Specific values for every variable (
The delay associated to RAN may be deducted in a very similar way. However, in this case we must consider a remote wireless user equipment and a base station with a C-RAN (Cloud-RAN) structure instead of the IP backbone and optical transport. It is important to note that, during handovers, although the core network does not participate in the process, the delay is modeled as a core network delay because remote base stations communicate with each other through an IP backbone.
The software modules participating in the communication process are divided into two groups: modules in the base station
As said before, in this model, all random variables without a specific distribution, are considered Gaussian, because of the central limit theorem.
Many different parameters in the proposed delay model are still unknown and will be evaluated later through model checking techniques to calculate a final probability distribution function for the end-to-end delay. In this process, the same model will be employed to evaluate the probability distribution of jitter, which is understood as the maximum expected delay fluctuation in normal conditions. The statistical analysis of the previously proposed model for the latency may also generate that result, if the standard deviation of partial probability distributions is calculated and combined (see Section 3.3 and Section 3.4). No additional model for jitter is then required.
Regarding network reliability, in this paper we focus on permanent effects (mainly the physical noise), instead of transient phenomena (such as electrical malfunctions). Basically, two main causes affect the network reliability in our model: congestion and physical noise. Congestion fills the queues of network elements rapidly, causing packets to be discarded as the system is blocked. In a M/M/1/N system, this blocking probability is calculated through the flow equilibrium Eq. (16), where
Physical noise is considered to follow a uniform distribution in the frequency domain, whose power
In the core network, all communications among routers in the IP backbone and/or among software modules belonging to the mobile network are independent events, and then, a global error probability
In RAN, congestion situations are usually caused by congestion in the base station not in the user devices (that only manage one communication link and serve only one user). Thus, modules where congestion may appear are those embedded into the 5G base stations. However, physical noise affects all devices equally. Then, the global error probability
Some of the previously presented parameters, such as physical noise, packet length, number of routers that are crossed by a packet, and optical fiber characteristics are contextual. As we perform evaluations using model checking techniques, we will consider existing information about the technological state of the art for the values of these parameters. On the other hand, parameters such as number of involved network modules, processing delays, and capacity of queues depend on the selected 5G network implementation. Many different proposals for core network and RAN scopes have been reported in the last years [11], but only some of them have evolved from the research world to production implementations. Thus, in this paper we have selected (for each network domain) the three most popular and developed solutions. So up to nine different network configurations may be defined. Specifically, we have selected ONAP [29], Free5GC [30], and Aether [31] for core network implementation; and ORAN [32], SD-RAN [33], OpenAirInterface for Radio Access Network (RAN) [34] implementation.
ONAP [29] is an opensource project focused on providing orchestration, management, and automation capabilities for network operators. By the time of writing, ONAP’s latest release was GUILIN (December 2020), which includes support for key 5G features such as network slicing, easy integration with ORAN networks, and native network functions. Free5GC [30] is a lighter but less automated and integrated solution. This project only provides containerized functionalities for each one of the modules composing a 5G core network (Network Slice Selection Function-NSSF-, Unified Data Management-UDM-, etc.). Aether [31] is a cloud-based “open-source” solution to build a network, compatible with 5G requirements and characteristics. Several authors and previous works claim this architecture is the most appropriate to implement 5G networks and, specially, URLLC. Therefore, it is worthy to be analyzed as well.
Regarding RAN implementations, ORAN (Open RAN) [32] is probably nowadays the most popular and advanced one. The main advantage of ORAN solutions is their low latency and processing delay. Besides is flexible enough to include new security modules and maintain a good performance. SD-RAN [33] is an opensource project based on the ORAN vision where basically, a near-real-time RAN Intelligent Controller (RIC) is provided. OpenAirInterface [34] is a project including open-source solutions for the RAN, core network, and Continuous Integration/Continuous Deployment (CI/CD) of 5G networks. Among these three proposals, the one focused on RAN is the most successful.
In our work, we assume all network implements follow the recommended default configuration. Moreover, while Aether is a monolithic architecture (so transmissions delays tend to be lower, although reliability tend to decrease); the other 5G implementations are distributed and many redundancies are considered (delays and jitter are higher, but reliability greatly improves). Finally, regarding hardware requirements, all the considered implementations have similar needs. Besides, delays introduced by hardware devices are exogenous variables and are independent from the structural feasibility analysis we are developing in this work. Future work will address this variable, but this initial study does not.
All described 5G implementations are open and then, they can be modified and enriched according to the users’ or service providers’ needs. Specifically, all of them allow the implementation of non-native security components to improve the global security level in URLLC. So, we can study network architectures and non-native security component independently.
Considering these different implementations and the way in which they operate (which network components they deploy, how they interact when stablishing a connection, etc.) we can define specific values, or a probability distribution, for variables in the previous models for delay, jitter, and reliability. During the experimental setup, the behavior of every network implementation (RAN or core) will be represented using a Markov model. Configurable network settings will be the variables of these models. The experiments will be based on this simulation framework (all possible scenarios will be considered thanks to a Monte Carlo approach) and no real network deployment will be required (see Section 3.3 and Section 4).
Tab. 1 summarizes all parameters from the proposed model together with some relevant information. Contextual parameters are those that do not depend on the network implementation (ONAP, ORAN, etc.), but on other variables such as the physical infrastructure. On the other hand, implementation specific parameters are those that are directly related to the selected network implementation.
3.2 Security Solutions in 5G Networks, Potential Attacks and Adversary Models
Cyber protection mechanisms can be divided into three different categories depending on the final objective: privacy modules, trust provision modules, and security modules [35]. Traditionally, mobile networks have employed a different classification (confidentiality, integrity, and availability) but fully secure URLLC must go further and cover not only network issues but also data security (integrity and privacy), fraud when using on-demand services, etc.
In general, 5G specifications include protocols and solutions to address most relevant challenges in all these areas, but for many application scenarios these native components are not enough [36]. In that way, non-native components enriching the performance at security level of 5G networks are essential [13]. In general, components described in the standards, such as the SUPI (Subscription Permanent Identifier) encryption, are already optimized to meet URLLC requirements. But when fully secure URLLC are considered, computationally heavier and more complex and slower non-native components are introduced. The feasibility of fully secure URLLC, then, is not guaranteed. Therefore, in this section we are focusing on non-native security components, not on standard solutions. In order to select the non-native components to be analyzed, we focus on those that have been employed, at least once, in a successful experience involving 5G networks (reported to the scientific world) in the last three years. Any component not meeting this condition is considered obsolete and is not included in this study.
Privacy technologies typically include anonymization and encryption solutions. Anonymization (and Pseudonymization) technologies process personal data so private information can no longer be attributed to a specific subject without a secret key. Most future commercial anonymization solutions for 5G are device-based (or SIM-based) and deployed in the endpoint. In this paper we consider the two most developed security proposals: Thales’ 5G SIM (Subscriber Identity Module) [37] and 5G Ensure Android OS [38]. 5G networks will be also provisioned with mechanisms to ensure the users’ privacy. These technologies are deployed in the core network [39]. Two very advanced mechanism will be considered in this work: 5G Ensure Enhanced Identity protection [40], and Ericsson Network Trace Anonymization [41]. In these anonymization solutions, although some management plane technologies would be essential, they have not been defined or reported yet. The most relevant attack against all these anonymization solutions is based on attackers performing analytic tasks. In this adversary model, an honest-but-curious analyst can observe the network traffic and traces. The objective of this adversary is to find all possible matches between personal information and protected private information circulating in the network. Previous studies have mathematically described this adversary model in detail [41].
The second group of privacy preserving technologies are cryptographic solutions. Almost every general-purpose cryptographic technology may be eventually applied to future 5G networks. However, in this paper, we focus on algorithms designed specifically for 5G networks or explicitly accepted by 3GPP to be used in that context. All these accepted algorithms are described in 3GPP technical reports TR33.841 [42]. Three basic technologies are considered in this paper: Radio interface encryption [43], Key Agreement protocols [44], and Key creation algorithms [45]. When encryption technologies are considered, the most relevant attack to be considered is the single-key attack [46]. This attack can be modeled as an adversary that assumes all packets from the same source are encrypted using the same private key (or a set of keys connected through simple relations). The objective of this adversary is to calculate the secret key and then, decrypt as many protected packets as possible. Many authors have proposed a formal and mathematical description of this adversary model [46].
Security components are, probably, the most developed non-native protection services in 5G networks nowadays. They include authentication services and integrity assurance solutions. Technical report T33.899 [47] defined by 3GPP describes the security aspects to be considered in next generation mobile networks. In this paper we consider two technologies: Technical report T33.501 from 3GPP [48], and IETF’s Perfect-Forward Secrecy for the Extensible Authentication Protocol Method for Authentication and Key Agreement (EAP-AKA′ PFS) [49]. Most relevant adversary models regarding these authentication technologies are focused on spoof attacks. In spoofing attacks, an adversary tries to successfully identify him/herself as another legitimate user by falsifying data, to gain an illegitimate advantage. The mathematical description for this adversary model can be found in several previous works [47].
Regarding integrity assurance mechanisms, technical report T33.501 also describes potential solutions for different contexts. In this paper, two basic technologies are analyzed: Non-access stratum (NAS) integrity algorithm, and Radio Resource Control (RRC) integrity mechanism and User Plane (UP) Packet Data Convergence Protocol (PDCP) [50]. In the proposed security analysis, the considered adversary model against integrity mechanisms is the standard data integrity threat. In this model, an adversary tries to modify or delete the content of data packets and corrupt the user communications. The adversary, in this case, knows the employed technologies and encryption schemes, as well as all relevant corporation information (except those information pieces that are managed as secret keys). A formal mathematical model for this adversary may be found in the literature [51].
Trust provision technologies are also envisioned to be integrated into 5G networks. Trust provision mechanisms include intrusion detection technologies and reputation management solutions. Two basic solutions for intrusion detection are considered in this paper: Sec5G IDPS (Intrusion detection and prevention system) [52] and SELFNET [53]. The most relevant attack performed by intruders is the sinkhole attack [54], in which the intruder tries to modify the system configuration to attract network traffic. This attack can be used to launch other attacks, like selective forwarding attack or acknowledge spoofing attack. In the state of the art, several mathematical models for this adversary may be found [55]. Reputation management solutions may include technological and social approaches. In general, these proposals are still being discussed at scientific level. Specifically, in this paper we are only considering one product: the SenderBase Reputation Service (SBRS) [56] provided by CISCOsystems. In the proposed security analysis, we consider as adversary model the self-promoting and slandering attacks, that both formally have the same mathematical and attacking model [57].
Apart from this objective-based classification, cyber protection solutions are usually classified according to the deployment scope. Three different deployment scopes can be defined in the context of 5G networks: core network, end-point devices, and management plane. The core network includes all modules and agents in charge of routing user traffic and providing services. End-point devices refer to all final and user equipment or infrastructure communicating through the core network. Finally, some components are not integrated within the user plane, but they are deployed in the management plane. These components are especially important in future 5G networks, where cloud solutions, remote dashboard, and Software Defined Networks (SDN) are envisioned to be exhaustively employed.
Tab. 2 shows a summary of all the considered cyber protection solutions, their scope, and their category. Additionally, the considered adversary models for further studies are provided as well. All the considered adversary models, types of attacks, attacker capabilities and countermeasures are well known threats, and (in this work) we are considering them as traditionally reported in the state of the art.
3.3 Formal Security Analysis Methodology
Model checking techniques focus on evaluating the probability of a system (described through a specification) to fulfill a certain property (described as a logic rule). Rules and specification may represent any possible scenario, situation, or stochastic process that must be evaluated. Specifically, if models represent a scenario where an adversary performs a known attack, and the property under study describes the success of that attack, the model checker generates a numerical function describing the probability of the attack to be successful. On the other hand, if the specification describes analytical models of a network, and the property under study represents the expected performance of the network, the model checker calculates the probability of a successful service provision with the expected Quality of Service. In this paper we use model checking techniques to evaluate the viability of secure URLLC for a given network configuration (see Section 3.1) and cyber protection solutions (see Section 3.2). To do that, models, tools, and expected QoS from 5G are set as described in the previous sections. For this analysis we used PRISM software [58] as model checker.
PRISM is a probabilistic model checker that enables the analysis of systems with a random behavior. It accepts several probabilistic models such as probabilistic automata and Markov decision processes. To match with the proposed probabilistic queue model based on Markov models and Poisson distributions, we model 5G networks as continuous-time Markov chains in this study. Models (specifications) must be described in PRISM language (a state-based language), while rules (properties) can be written using several languages (such as Linear Temporal Logic Rules or Continuous-time Stochastic Logic rules). In this study we use probabilistic CTL (continuous time logic) rules.
We created the specifications by combining the previously described analytical models with the attacker models and the models describing the behavior of each one of the described cyber protection solutions. On the other hand, we prepared properties to analyze the success of cyber-attacks and the probability of delay, jitter, and reliability to show the expected values of 5G URLLC.
We performed our analysis in a Linux-based machine (Ubuntu 18.04 LTS) with the following hardware characteristics: Dell R540 server with 96 GB of RAM, two processors Intel Xeon Silver 4114 2.2G, and a HD 2TB SATA 7, 2K rpm hard drive. As results may be affected by hardware phenomena and numerical errors, each analysis was repeated 12 times. The final results employed for further analyses were calculated as the mean value of all partial calculations.
With this simulation strategy, we guarantee the experimental error is below 10% (error is one magnitude order lower than the results). According to the error theory, when final results are calculated as the average value of a set of samples, the relative error is inversely proportional to the number of samples (25). For twelve samples, experimental error is around 8%.
3.4 Stochastic Cost-Security Analysis
PRISM model checker generates a probability density function for each rule introduced in the system. Besides, the probability of all (or a subset of) rules being met can also be calculated. However, deeper analyses are required for our study. The basic challenge we face is to analyze the risk or security reduction we are willing to accept in order to ensure the performance of URLLC or, equally, what kind of balances between both important issues are feasible and may be supported at long-term. To do that, results related to network performance and URLLC viability must be combined using the adequate cost functions.
From PRISM model checker we obtain a set of A probabilities
For each of these probabilities, we define a threshold
Considering the analytical models (see Section 3.1) and the requirements of URLLC in 5G networks (see Section 1), we define three additional relevant probabilities describing the viability of URLLC [5]:
• The probability
• The probability
• The probability
We propose a cost function to calculate a global evaluation of all these factors together. In its general form, the cost function
Many different functions may be defined to meet the previously described conditions. As the complexity of attacks and cyber risks to be represented increases, the mathematical complexity of the cost function also increases. In this first work, in order to build the stochastic function, different qualitative facts are taken into account. Namely:
• Networks’ performance and user traffic follow exponential laws. In general, above a certain threshold, the expected behavior from networks gets worse very fast, typically exponentially.
• In most standard services, QoS is more affected by high jitter values than by high, and stable, delay values. In general, users and applications can tolerate a delay, but relevant fluctuations in time are difficult to manage.
• Equally, reliability is a more relevant component of 5G QoS than latency. Packet losses may prevent the communication services, while high delay may be managed although the QoS decreases.
• All attacks are, in general, independent, and therefore their success probabilities are also totally independent. Although, in some cases, attacks are interconnected and cross-layer, and may be directed against several network modules at the same time; in this first work we are addressing the basic attacker models (see Tab. 2), which are focused on only one objective and target. Future works will consider more complicated attacking schemes.
• The value of a vulnerable network configuration is negligible. Thus, the global value must be null if any success probability
With all previous consideration we propose a cost function
The proposed cost function may be understood as a measure of the success rate of secure URLLC, for a given network configuration. While calculating the different probabilities that compose this model, as they are random phenomena, we have some uncertainties to quantify. This is called the significance level. Specifically, the significance level is understood as the probability of the proposed cost function to consider URLLC feasible when they are not. This value may be also understood as a measure of how precise our calculations are.
Considering this cost function, the methodology for security analyses based on PRISM model checker (Section 3.3), and the analytical models (Section 3.1), we perform a global evaluation of security mechanisms in the context of different network configuration. To perform this final and global analysis, we introduced partial data in MATLAB 2018b software for processing and visualization, running on an Ubuntu 18.04 LTS system.
As many variables may affect the results of this study, we consider the following simplifications: first, we fix all significance levels
At this point, we must remember the proposed cost function may be understood as a measure of the success rate of secure URLLC, for a given network configuration. And the significance level may be understood as a measure of how precise our calculations are (as lower it is, the global error is lower). Specifically, the global significance level
Computing the cost of all considered network architectures (see Section 3.1), when no protection mechanisms are implemented or analyzed, the architecture based on ONAP and ORAN technologies is the one showing the best performance, supporting URLLC in a successful manner in 98% of cases. On the contrary, SD-RAN technology is always associated to a lower performance, and only 89% of cases URLLC is successfully supported. In further analyses, these percentages are the reference to conclude whether cyber protection has a relevant impact or not in URLLC.
For the rest of this study, we focus on the architecture that showed the best performance (i.e., ONAP as core implementation and ORAN as RAN technology). Fig. 1a shows the global cost of the core network cyber protection solutions described in Section 3.2 (see Tab. 1) in the context of this architecture and for different significance levels, while Fig. 1b shows the global cost (and URLLC viability) when different combinations of these elements are deployed.
Figure 1: Global cost of cyber protection on ONAP+ORAN implementation
Fig. 1b show that trust-based solutions affect URLLC the least, because trust provision mechanisms operate in parallel to communication services. Therefore, they do not mutually affect each other. We can also observe that the considered significance levels produce small variations on the global cost. On the contrary, authentication-(the most) and cryptographic-and anonymization-based solutions (more slightly) highly affect the global cost, causing a reduction up to 30% compared to the baseline. For these mechanisms, the dynamic and periodical calculation of keys and/or authentication algorithms introduces a significant and variable delay which highly increases jitter (going above the limit envisioned for 5G networks). Thus, only 60% (approximately) of cases represent a successful URLLC provision. As the significance level increases, security requirements get relaxed, and the global cost increases as well. However, the system is less secure. A balance may be reached only if a 65% success in provisioning URLLC is assumed. A similar but less critical situation is observed regarding integrity mechanisms. Although they introduce a non-negligible delay, they also increase the network reliability. Thus, the global cost, although reduced, maintains in a more stable value with respect to the baseline (around a successful URLLC provision rate of 85%). In general, and only considering core security solutions, a global cyber-protected 5G network can only guarantee a 60% of success in URLLC provision (KEY FINDING#1). Although an official success rate threshold in which URLLC are not considered feasible has not been defined, it is clear that URLLC is already highly affected under existing architectures and solutions. This finding also answers the second research question (REQ#2), and we can conclude secure 5G URLLC are not feasible in core networks.
Fig. 1c shows the global cost of endpoint cyber protection mechanisms for the ONAP and ORAN architecture when no core cyber protection solutions are deployed, while Fig. 1d shows the global cost when a combination of different endpoint cyber protection solutions are considered (see Tab. 1).
The general behavior, in this case, is similar to the one observed in core security mechanisms. In this case, as the number of elements and modules communicating is lower (only the user device and the base station is considered), the impact of security solution is less relevant. Privacy mechanisms for end devices are embedded into the SIM card or the mobile operating system, so they do not affect the network performance. Only small variations caused by the different significance level are observed in privacy instruments. On the contrary, security (and particularly authentication) solutions introduce a relevant jitter and delay that highly affect the URLLC feasibility and provision as introduce traffic in the network. In particular, a scheme in which endpoint cyber protection solutions are deployed only guarantees a success probability of 65% (approximately) for URLLC (KEY FINDING#2). Similar to core technology cyber protections, in this case it is clear that URLLC is also highly affected. With this finding, we can also answer the third research question (REQ#3). And we can conclude URLLC are not feasible if 5G endpoints implement currently existing security mechanisms.
We conduct the same analysis for management plane solutions (see Fig. 1e) in isolation (i.e., no core or endpoint cyber protection solutions deployed). In this case, as management operations are sporadic, they rarely affect standard communication services and URLLC. Some impact in jitter can be observed as these operations introduce a relevant and non-constant delay. However, as these operations are very sporadic, their effect is diluted in long stable periods, meeting the URLLC jitter requirements. As can be seen, URLLC is feasible with a probability around 90%, so we can conclude the impact of management plane security and privacy mechanisms in URLLC is negligible (KEY FINDING#3).
Fig. 1f shows the results for all network segments (endpoints, core, and management plane), considering all cyber protection mechanisms. As in all previous analyses, trust provision technologies (that are only present in the core network) do not affect the URLLC performance, but security and privacy mechanisms have a relevant impact. Specifically, security technologies that include authentication solutions have a significant impact in jitter (one key and very important parameter in 5G URLLC).
Globally, a fully secure 5G network (based on ONAP and ORAN) can only guarantee URLLC with a probability of 55% (approximately). As a conclusion, secure URLLC cannot be guaranteed using the best current solutions together (KEY FINDING#4), but a new optimization or more efficient cyber protection schemes may reach that objective, as technically URLLC is feasible with a 55% success rate.
This last finding finally answers the first research question (REQ#1). We can conclude the current technological state does not make feasible secure 5G URLLC.
For completeness, we conducted the same analysis with architectures based on ONAP as core implementation but considering SD-RAN and OpenAirInterface for the RAN. Fig. 2 shows the results considering all network segments (i.e., endpoint, core, and management plane).
Figure 2: Global cost (all subsystems) of cyber protection solutions in ONAP+SD-RAN (left) and ONAP+OpenAirInterface (right) architectures
Fig. 2 shows the same behavior observed for ORAN, although the global and final values are significantly lower. Specifically, networks based on ONAP and SD-RAN architectures may only support secure URLLC with a 45% success; while for ONAP and OpenAirInterface architectures success rate goes up to 53% (approximately). The same conclusion than for previous architectures may be extracted in this case. In general, secure URLLC are not feasible with current architectures and cyber protection mechanisms. However, technologies have the potential to reach that point, as showed by the success rates around 50%.
For the remaining network architectures, as the observed evolution for different significance levels is independent of the network configuration, we only focus on cost calculated for a significance level of 0.1 so, results can be displayed in a clearer manner.
Fig. 3 (left) shows the obtained results for architectures based on Free5GC, while Fig. 3 (right) shows the results for networks based on Aether core networks.
Figure 3: Global cost of solutions in Free5GC-based (left) and Aether-based (right) architectures
For all cases, the cost (or success rate) for secure URLLC is around 50%, being slightly lower (45%, approximately) for SD-RAN networks and slightly higher (around 55%) for ORAN deployments. From these results it can be deducted that the underlaying network architecture only affects global cost in a small percentage. In general, the impact of jitter and delays introduced by cyber protection mechanisms are larger than the differences initially observed among the different network configurations.
Thus, as main and global conclusion, secure URLLC are not feasible with current 5G implementations and cyber protection mechanisms. New and more efficient cyber protection strategies and schemes are required and should be studied.
Threats to the internal validity are not relevant in our results. PRISM model checker considers statistical methods to reduce the impact of bias and numerical error or malfunctions. Besides, standard software, systems and libraries were employed in order to avoid calibration or instrumentation problems.
Regarding the external validity, numerical results obtained through the proposed cost function may change if different mathematical functions are employed in the future (in order to represent more complex attacks) but conclusions will remain the same. Actually, and according to the Taylor series theory (35), the proposed scenario (where attacks are independent) is always a better case than cross-layer attacks. Any generic (cost) function
5 Conclusions, Limitations, Future Work and Networks Beyond 5G
In this work we introduce a cost-security function as a method to evaluate the performance and adequacy of security mechanisms in 5G networks to support ultra-reliable low-latency communications (URLLC). We categorized existing security solutions according to their purpose and deployment scope. Each one is analyzed in the context of different 5G architecture implementations using two different approaches. First, using model checking techniques, we evaluated the probability of an attacker to be successful against each security technology. Second, using analytical models, we analyzed the impact of these security mechanisms in terms of delay, throughput consumption, and reliability. Finally, we combined both analyses using stochastic cost-security functions and the PRISM model checker to create a global picture.
Our simulation results show how cyber protection mechanisms have a relevant impact in URLLC. Precisely, a fully secure 5G network can only guarantee URLLC with a probability of ∼55% using the best configuration of available core and RAN technologies. Furthermore, it may turn even unfeasible to provide secure URLLC in some scenarios and 5G implementations.
Four key findings are presented in this work:
• In general, and only considering core security solutions, a global cyber-protected 5G network can only guarantee a 60% of success in URLLC provision.
• A scheme in which endpoint cyber protection solutions are deployed only guarantees a success probability of 65% (approximately) for URLLC.
• The impact of management plane security and privacy mechanisms in URLLC is negligible.
• Secure URLLC cannot be guaranteed using the best current solutions together.
The proposed analysis is valid for network deployments with a homogenous composition. In our framework, the RAN, and the core network, are based on one unique technology with a standard and known behavior. The applicability of our proposal to transition scenarios is limited, as hybrid technologies are typically employed and different values for delays, jitter and packet losses are observed in different network domains. On the other hand, the proposed cost-security functions are only valid for consumer applications. Other scenarios such as critical infrastructures, emergency systems, military communications, etc. show very specific QoS requirements and, eventually, the proposed stochastic cost-security functions should be adapted to represent in a better way the real weight of every factor in those scenarios.
Our future work will consider real network deployments and real cyber protection mechanisms to carry out experiments that show the actual performance of secure URLLC in practice.
Finally, the proposed framework is also valid for networks beyond 5G, such as the novel 6G networks. In general, mobile networks beyond 5G show similar behavior and QoS requirements to current 5G schemes. Although some changes could be done at physical level (RAN and core network), most important changes are envisioned to affect the network and service management solutions. Our approach perfectly fits these future networks as well, as different management protocols or service provision architectures can be considered just by introducing the right value for the number of interconnected modules in the RAN and core network, the new physical characteristics of networks and the actual description of the network topology.
Funding Statement: The publication is produced within the framework of Ramón Alcarria y Borja Bordel’s research projects on the occasion of their stay at Argonne Labs (José Castillejo’s 2021 grant). This work is supported by the Ministry of Science, Innovation and Universities through the COGNOS project.
Conflicts of Interest: The authors declare that they have no conflicts of interest to report regarding the present study.
References
- M. Shafi, A. F. Molisch, P. J. Smith, T. Haustein, P. Zhu et al., “5G: A tutorial overview of standards, trials, challenges, deployment, and practice,” IEEE Journal on Selected Areas in Communications, vol. 35, no. 6, pp. 1201–1221, 2017.
- B. Bordel, R. Alcarria, T. Robles and D. González, “An industry 4.0 solution for the detection of dangerous situations in civil work scenarios,” in Int. Conf. on Information Technology & Systems, Springer, Cham, pp. 494–504 2019.
- B. Bordel, R. Alcarria, T. Robles and D. Martín, “Cyber–physical systems: Extending pervasive sensing from control theory to the internet of things,” Pervasive and Mobile Computing, vol. 40, pp. 156–184, 2017.
- H. Gamage, N. Rajatheva and M. Latva-Aho, “Channel coding for enhanced mobile broadband communication in 5G systems,” in 2017 European Conf. on Networks and Communications (EuCNC), Oulu, Finland, IEEE, pp. 1–6, 2017.
- G. Pocovi, H. Shariatmadari, G. Berardinelli, K. Pedersen, J. Steiner et al., “Achieving ultra-reliable low-latency communications: Challenges and envisioned system enhancements,” IEEE Network, vol. 32, no. 2, pp. 8–15, 2018.
- C. Bockelmann, N. Pratas, H. Nikopour, K. Au, T. Svensson et al., “Massive machine-type communications in 5G: Physical and MAC-layer solutions,” IEEE Communications Magazine, vol. 54, no. 9, pp. 59–65, 201
- S. Nowaczewski and W. Mazurczyk, “Securing future internet and 5G using customer edge switching using DNSCrypt and DNSSEC,” Journal of Wireless Mobile Networks, Ubiquitous Computing, and Dependable Applications, vol. 11, no. 3, pp. 87–106, 2020.
- B. Bordel, R. Alcarria, T. Robles and A. Sánchez-Picot, “Stochastic and information theory techniques to reduce large datasets and detect cyberattacks in ambient intelligence environments,” IEEE Access, vol. 6, pp. 34896–34910, 201
- B. Bordel, A. B. Orúe, R. Alcarria and D. Sánchez-De-Rivera, “An intra-slice security solution for emerging 5G networks based on pseudo-random number generators,” IEEE Access, vol. 6, pp. 16149–16164, 2018.
- A. Gupta and R. K. Jha, “A survey of 5G network: Architecture and emerging technologies,” IEEE Access, vol. 3, pp. 1206–1232, 2015.
- J. M. Hamamreh, E. Basar and H. Arslan, “OFDM-Subcarrier index selection for enhancing security and reliability of 5G URLLC services,” IEEE Access, vol. 5, pp. 25863–25875, 2017.
- J. Cao, M. Ma, H. Li, R. Ma, Y. Sun et al., “A survey on security aspects for 3GPP 5G networks,” IEEE Communications Surveys & Tutorials, vol. 22, no. 1, pp. 170–195, 2020.
- R. Marin-Perez, D. Garcia-Carrillo, J. Sanchez-Gomez and A. Skarmeta, “EAP-Based bootstrapping for secondary service authentication to integrate IoT into 5G networks,” in Mobile Internet Security: 4th Int. Symp., MobiSec 2019, Taichung, Taiwan, Springer Nature, vol. 1121, pp. 13, 2020.
- I. Ahmad, T. Kumar, M. Liyanage, J. Okwuibe, M. Ylianttila et al., “5G security: Analysis of threats and solutions,” in 2017 IEEE Conf. on Standards for Communications and Networking (CSCN), Helsinki, Finland, IEEE, pp. 193–199, 2017.
- T. Yoshizawa, S. B. M. Baskaran and A. Kunz, “Overview of 5G URLLC system and security aspects in 3GPP,” in 2019 IEEE Conf. on Standards for Communications and Networking (CSCN), Granada, Spain, IEEE, pp. 1–5, 2019.
- R. Chen, C. Li, S. Yan, R. Malaney and J. Yuan, “Physical layer security for ultra-reliable and low-latency communications,” IEEE Wireless Communications, vol. 26, no. 5, pp. 6–11, 2019.
- H. Ren, C. Pan, Y. Deng, M. Elkashlan and A. Nallanathan, “Resource allocation for secure URLLC in mission-critical IoT scenarios,” IEEE Transactions on Communications, vol. 68, no. 9, pp. 5793–5807, 2020.
- F. Al-Turjman, “Intelligence and security in big 5G-oriented IoNT: An overview,” Future Generation Computer Systems, vol. 102, pp. 357–368, 2020.
- S. Gallenmüller, J. Naab, I. Adam and G. Carle, “5G QoS: Impact of security functions on latency,” in NOMS 2020–2020 IEEE/IFIP Network Operations and Management Symp., Budapest, Hungary, IEEE, pp. 1–9, 2020.
- P. Emmerich, D. Raumer, A. Beifuß, L. Erlacher, F. Wohlfart et al., “Optimizing latency and CPU load in packet processing systems,” in 2015 Int. Symp. on Performance Evaluation of Computer and Telecommunication Systems (SPECTS), Chicago, USA, IEEE, pp. 1–8, 2015.
- A. Herdrich, E. Verplanke, P. Autee, R. Illikkal, C. Gianos et al., “Cache QoS: From concept to reality in the intel® xeon® processor E5–2600 v3 product family,” in 2016 IEEE Int. Symp. on High Performance Computer Architecture (HPCA), Barcelona, Spain, IEEE, pp. 657–668, 2016.
- D. Fang and Y. Qian ““5G wireless security and privacy: Architecture and flexible mechanisms,” IEEE Vehicular Technology Magazine, vol. 15, no. 2, pp. 58–64, 2020.
- K. Jeong, H. Kang, C. Lee, J. Sung, S. Hong et al., “Weakness of lightweight block ciphers mCrypton and LED against biclique cryptanalysis,” Peer-to-Peer Networking and Applications, vol. 8, no. 4, pp. 716–732, 2015.
- D. Rupprecht, K. Kohls, T. Holz and C. Pöpper, “Breaking LTE on layer two,” in 2019 IEEE Symp. on Security and Privacy (SP), San Francisco, USA, IEEE, pp. 1121–1136, 2019.
- K. Kohls, D. Rupprecht, T. Holz and C. Pöpper, “Lost traffic encryption: Fingerprinting lte/4G traffic on layer two,” in Proc. of the 12th Conf. on Security and Privacy in Wireless and Mobile Networks, Miami, USA, pp. 249–260, 2019.
- H. Kim, J. Lee, E. Lee and Y. Kim, “Touching the untouchables: Dynamic security analysis of the LTE control plane,” in 2019 IEEE Symp. on Security and Privacy (SP), San Francisco, USA, IEEE, pp. 1153–1168, 2019.
- S. R. Hussain, M. Echeverria, O. Chowdhury, N. Li and E. Bertino, “Privacy attacks to the 4G and 5G cellular paging protocols using side channel information,” Network and Distributed Systems Security (NDSS) Symposium, San Diego, USA, pp. 1–15, 2019.
- 3rd Generation Partnership Project, “Technical specification group services and system aspects. release 15 description.” Online: https://www.3gpp.org/release-15. 2019.
- V. Q. Rodriguez, R. Corbel, F. Guillemin and A. Ferrieux, “Cloud-RAN factory: Instantiating virtualized mobile networks with ONAP,” in 2020 11th Int. Conf. on Network of the Future (NoF), Bordeaux, France, IEEE, pp. 120–122, 2020.
- L. Tomaszewski, S. Kukliński and R. Kołakowski, “A new approach to 5G and MEC integration,” in IFIP Int. Conf. on Artificial Intelligence Applications and Innovations, Springer, Cham, pp. 15–24, 2020.
- Aether, “Private 4G/5G connected edge platform for enterprises,” Online: https://aetherproject.org/wp-content/uploads/2020/12/Aether-White-Paper-Dec2020.pdf. 2020.
- I. Chih-Lin and S. Katti, “O-RAN: Towards an open and smart RAN,” O-RAN ALLIANCE White Paper. October 2018. Online: https://www.o-ran.org/s/O-RAN-WP-FInal-181017.pdf.
- Z. Zaidi, V. Friderikos and M. A. Imran, “Future RAN architecture: SD-RAN through a general-purpose processing platform,” IEEE Vehicular Technology Magazine, vol. 10, no. 1, pp. 52–60, 2015.
- N. Nikaein, M. K. Marina, S. Manickam, A. Dawson, R. Knopp et al., “Openairinterface: A flexible platform for 5G research,” ACM SIGCOMM Computer Communication Review, vol. 44, no. 5, pp. 33–38, 2014.
- B. Bordel, R. Alcarria, D. M. De Andrés and I. You, “Securing internet-of-things systems through implicit and explicit reputation models,” IEEE Access, vol. 6, pp. 47472–47488, 2018.
- B. Bordel, R. Alcarria, T. Robles and M. S. Iglesias, “Data authentication and anonymization in IoT scenarios and future 5G networks using chaotic digital watermarking,” IEEE Access, vol. 9, pp. 22378–22398, 2021.
- M. Pauliac, “USIM in 5G Era,” Journal of ICT Standardization, vol. 8, no. 1, pp. 29–39, 2020.
- N. Paladi and L. Karlsson, “Deliverable D3. 8 5G-PPP security enablers documentation (v2. 0) enabler BootstrappingTrust,” 5G Enablers for Network and System Security and Resilience Project, vol. 2017, pp. 1–190, 2017.
- H. Kim, “5G core network security issues and attack classification from network protocol perspective,” Journal of Internet Services and Information Security, vol. 10, no. 2, pp. 1–15, 2020.
- J. Hiltunen, O. Mammela, P. Ruuska, J. Suomalainen, P. Bisson et al., “5G-ENSURE-D3. 2 5G-PPP security enablers open specifications (v1.0),” 5G Enablers for Network and System Security and Resilience Project, vol. 2016, pp. 1–190, 2016.
- M. Mohammady, L. Wang, Y. Hong, H. Louafi, M. Pourzandi et al., “Preserving both privacy and utility in network trace anonymization,” in Proc. of the 2018 ACM SIGSAC Conf. on Computer and Communications Security, pp. 459–474, 2018.
- Study on the support of 256-bit algorithms for 5G,” 3GPP TR 33.841, March 2017.
- A. Abdullah, “Advanced encryption standard (AES) algorithm to encrypt and decrypt data,” Cryptography and Network Security, vol. 16, pp. 1–12, 2017.
- J. H. Yoo, H. U. Kim and Y. H. Jung, “A design of MILENAGE algorithm-based mutual authentication protocol for the protection of initial identifier in LTE,” Journal of Venture Innovation, vol. 2, no. 1, pp. 13–21, 2019.
- F. Chen and J. Yuan, “Enhanced key derivation function of HMAC-SHA-256 algorithm in LTE network,” in Proc. of the 2012 Fourth Int. Conf. on Multimedia Information Networking and Security, Nanjin,China, pp. 15–18, 2012.
- O. Dunkelman, N. Keller and A. Shamir, “Improved single-key attacks on 8-round AES-192 and AES-256,” in Int. Conf. on the Theory and Application of Cryptology and Information Security, Springer, Berlin, Heidelberg, pp. 158–176, 2010.
- Study on the security aspects of the next generation system,” 3GPP TR 33.899, 2017.
- Security architecture and procedures for 5G system,” 3GPP TS 33.501, March 2017.
- J. Arkko, K. Norrman and V. Torvinen, “Perfect-forward secrecy for the extensible authentication protocol method for authentication and key agreement (EAP-AKA′ PFS),” IETF, vol. 2019, pp. 1–25, January 2019.
- 3GPP TS 33.401. “3GPP system architecture evolution (SAE); security architecture,” 2016.
- Z. Zhang, Y. Wang and L. Xie, “A novel data integrity attack detection algorithm based on improved grey relational analysis,” IEEE Access, vol. 6, pp. 73423–73433, 2018.
- Sec5g: Securing 5G for mission critical services,” OneSource, Consultoria Informáica Lda. Online: https://5ginfire.eu/sec5g/. 2020.
- SELFNET, “Deliverable D2.1: Use cases definition and requirements of the systems and its components,” October 2015. Online: https://bscw.selfnet-5g.eu/pub/bscw.cgi/d18783/SELFNET%20Deliverable%202.1%20-%20Final%20v12.pdf.
- A. U. Rehman, S. U. Rehman and H. Raheem, “Sinkhole attacks in wireless sensor networks: A survey,” Wireless Personal Communications, vol. 106, no. 4, pp. 2291–2313, 2019.
- C. Cervantes, D. Poplade, M. Nogueira and A. Santos, “Detection of sinkhole attacks for supporting secure routing on 6LoWPAN for internet of things,” in 2015 IFIP/IEEE Int. Symp. on Integrated Network Management (IM), Ottawa, Canada, IEEE, pp. 606–611, 2015.
- J. Porenta and M. Ciglarič, “Empirical comparison of IP reputation databases,” in Proc. of the 8th Annual Collaboration, Electronic Messaging, Anti-Abuse and Spam Conf., Perth, Australia, pp. 220–226, 2011.
- K. Hoffman, D. Zage and C. Nita-Rotaru, “A survey of attack and defense techniques for reputation systems,” ACM Computing Surveys (CSUR), vol. 42, no. 1, pp. 1–31, 2009.
- M. Kwiatkowska, G. Norman and D. Parker, “PRISM 4.0: Verification of probabilistic real-time systems,” in Int. Conf. on Computer Aided Verification, Springer, Berlin, Heidelberg, pp. 585–591, 2011.
Cite This Article
B. Bordel, R. Alcarria, J. Chung, R. Kettimuthu, T. Robles et al., "Towards fully secure 5g ultra-low latency communications: a cost-security functions analysis," Computers, Materials & Continua, vol. 74, no.1, pp. 855–880, 2023. https://doi.org/10.32604/cmc.2023.026787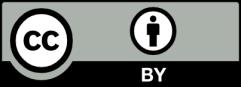