Open Access
ARTICLE
Real-Time Multiple Guava Leaf Disease Detection from a Single Leaf Using Hybrid Deep Learning Technique
1 Department of CS&SE, Islamic International University, Islamabad, 44000, Pakistan
2 Department of IT Services, University of Okara, Okara, 56310, Pakistan
3 Department of CS, University of Okara, Okara, 56310, Pakistan
4 Department of Botany, University of Okara, Okara, 56310, Pakistan
5 Department of Computer, College of Science and Arts in Ar Rass, Qassim University, Ar Rass, Qassim, 52571, Saudi Arabia
* Corresponding Author: Tamim Alkhalifah. Email:
Computers, Materials & Continua 2023, 74(1), 1235-1257. https://doi.org/10.32604/cmc.2023.032005
Received 03 May 2022; Accepted 22 June 2022; Issue published 22 September 2022
Abstract
The guava plant has achieved viable significance in subtropics and tropics owing to its flexibility to climatic environments, soil conditions and higher human consumption. It is cultivated in vast areas of Asian and Non-Asian countries, including Pakistan. The guava plant is vulnerable to diseases, specifically the leaves and fruit, which result in massive crop and profitability losses. The existing plant leaf disease detection techniques can detect only one disease from a leaf. However, a single leaf may contain symptoms of multiple diseases. This study has proposed a hybrid deep learning-based framework for the real-time detection of multiple diseases from a single guava leaf in several steps. Firstly, Guava Infected Patches Modified MobileNetV2 and U-Net (GIP-MU-NET) has been proposed to segment the infected guava patches. The proposed model consists of modified MobileNetv2 as an encoder, and the U-Net model’s up-sampling layers are used as a decoder part. Secondly, the Guava Leaf Segmentation Model (GLSM) is proposed to segment the healthy and infected leaves. In the final step, the Guava Multiple Leaf Diseases Detection (GMLDD) model based on the YOLOv5 model detects various diseases from a guava leaf. Two self-collected datasets (the Guava Patches Dataset and the Guava Leaf Diseases Dataset) are used for training and validation. The proposed method detected the various defects, including five distinct classes, i.e., anthracnose, insect attack, nutrition deficiency, wilt, and healthy. On average, the GIP-MU-Net model achieved 92.41% accuracy, the GLSM gained 83.40% accuracy, whereas the proposed GMLDD technique achieved 73.3% precision, 73.1% recall, 71.0% mAP@0.5 and 50.3 mAP@0.5:0.95 scores for all the aforesaid classes.Keywords
Cite This Article
J. Rashid, I. Khan, G. Ali, S. U. Rehman, F. Alturise et al., "Real-time multiple guava leaf disease detection from a single leaf using hybrid deep learning technique," Computers, Materials & Continua, vol. 74, no.1, pp. 1235–1257, 2023. https://doi.org/10.32604/cmc.2023.032005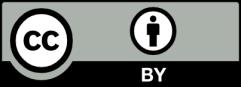