Open Access
ARTICLE
A Hybrid Deep Fused Learning Approach to Segregate Infectious Diseases
1 Department of Software Engineering, Nisantasi University, Istanbul, 34398, Turkey
2 Dapartment of Computer Science, College of Computer Engineering and Sciences in Al-Kharj, Prince Sattam Bin Abdulaziz University, P.O. Box 151, Al-Kharj, 11942, Saudi Arabia
* Corresponding Author: Jawad Rasheed. Email:
Computers, Materials & Continua 2023, 74(2), 4239-4259. https://doi.org/10.32604/cmc.2023.031969
Received 02 May 2022; Accepted 01 September 2022; Issue published 31 October 2022
Abstract
Humankind is facing another deadliest pandemic of all times in history, caused by COVID-19. Apart from this challenging pandemic, World Health Organization (WHO) considers tuberculosis (TB) as a preeminent infectious disease due to its high infection rate. Generally, both TB and COVID-19 severely affect the lungs, thus hardening the job of medical practitioners who can often misidentify these diseases in the current situation. Therefore, the time of need calls for an immediate and meticulous automatic diagnostic tool that can accurately discriminate both diseases. As one of the preliminary smart health systems that examine three clinical states (COVID-19, TB, and normal cases), this study proposes an amalgam of image filtering, data-augmentation technique, transfer learning-based approach, and advanced deep-learning classifiers to effectively segregate these diseases. It first employed a generative adversarial network (GAN) and Crimmins speckle removal filter on X-ray images to overcome the issue of limited data and noise. Each pre-processed image is then converted into red, green, and blue (RGB) and Commission Internationale de l’Elcairage (CIE) color spaces from which deep fused features are formed by extracting relevant features using DenseNet121 and ResNet50. Each feature extractor extracts 1000 most useful features which are then fused and finally fed to two variants of recurrent neural network (RNN) classifiers for precise discrimination of three-clinical states. Comparative analysis showed that the proposed Bi-directional long-short-term-memory (Bi-LSTM) model dominated the long-short-term-memory (LSTM) network by attaining an overall accuracy of 98.22% for the three-class classification task, whereas LSTM hardly achieved 94.22% accuracy on the test dataset.Keywords
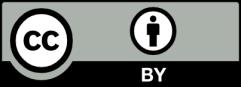