Open Access
ARTICLE
Network Intrusion Detection Based on Feature Selection and Hybrid Metaheuristic Optimization
1 Department of Information Technology, College of Computer and Information Sciences, Princess Nourah bint Abdulrahman University, P.O. Box 84428, Riyadh, 11671, Saudi Arabia
2 Department of Communications and Electronics, Delta Higher Institute of Engineering and Technology, Mansoura, 35111, Egypt
3 Department of Computer Science, Faculty of Computer and Information Sciences, Ain Shams University, Cairo, 11566, Egypt
4 Department of Computer Science, College of Computing and Information Technology, Shaqra University, 11961, Saudi Arabia
5 Computer Engineering and Control Systems Department, Faculty of Engineering, Mansoura University, Mansoura, 35516, Egypt
6 Information Systems Department, College of Computer and Information Sciences, Princess Nourah bint Abdulrahman University, P.O. Box 84428, Riyadh, 11671, Saudi Arabia
7 Department of System Programming, South Ural State University, Chelyabinsk, 454080, Russia
8 Department of Computer Sciences, College of Computer and Information Sciences, Princess Nourah bint Abdulrahman University, P.O. Box 84428, Riyadh, 11671, Saudi Arabia
* Corresponding Author: Doaa Sami Khafaga. Email:
Computers, Materials & Continua 2023, 74(2), 2677-2693. https://doi.org/10.32604/cmc.2023.033273
Received 13 June 2022; Accepted 01 August 2022; Issue published 31 October 2022
Abstract
Applications of internet-of-things (IoT) are increasingly being used in many facets of our daily life, which results in an enormous volume of data. Cloud computing and fog computing, two of the most common technologies used in IoT applications, have led to major security concerns. Cyberattacks are on the rise as a result of the usage of these technologies since present security measures are insufficient. Several artificial intelligence (AI) based security solutions, such as intrusion detection systems (IDS), have been proposed in recent years. Intelligent technologies that require data preprocessing and machine learning algorithm-performance augmentation require the use of feature selection (FS) techniques to increase classification accuracy by minimizing the number of features selected. On the other hand, metaheuristic optimization algorithms have been widely used in feature selection in recent decades. In this paper, we proposed a hybrid optimization algorithm for feature selection in IDS. The proposed algorithm is based on grey wolf (GW), and dipper throated optimization (DTO) algorithms and is referred to as GWDTO. The proposed algorithm has a better balance between the exploration and exploitation steps of the optimization process and thus could achieve better performance. On the employed IoT-IDS dataset, the performance of the proposed GWDTO algorithm was assessed using a set of evaluation metrics and compared to other optimization approaches in the literature to validate its superiority. In addition, a statistical analysis is performed to assess the stability and effectiveness of the proposed approach. Experimental results confirmed the superiority of the proposed approach in boosting the classification accuracy of the intrusion in IoT-based networks.Keywords
Cite This Article
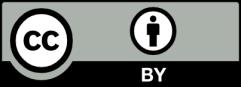