Open Access
ARTICLE
Reducing Dataset Specificity for Deepfakes Using Ensemble Learning
1 Faculty of Computer and Information Systems Islamic University Madinah, Madinah, 42351, Saudi Arabia
2 Department of Computer Science, Lahore Garrison University, Lahore, 54000, Pakistan
3 The University of Lahore, Sargodha, 40100, Pakistan
4 University of Sargodha, Department of Computer Science & IT, Sargodha, 40100, Pakistan
* Corresponding Author: Tahir Alyas. Email:
Computers, Materials & Continua 2023, 74(2), 4261-4276. https://doi.org/10.32604/cmc.2023.034482
Received 18 July 2022; Accepted 07 September 2022; Issue published 31 October 2022
Abstract
The emergence of deep fake videos in recent years has made image falsification a real danger. A person’s face and emotions are deep-faked in a video or speech and are substituted with a different face or voice employing deep learning to analyze speech or emotional content. Because of how clever these videos are frequently, Manipulation is challenging to spot. Social media are the most frequent and dangerous targets since they are weak outlets that are open to extortion or slander a human. In earlier times, it was not so easy to alter the videos, which required expertise in the domain and time. Nowadays, the generation of fake videos has become easier and with a high level of realism in the video. Deepfakes are forgeries and altered visual data that appear in still photos or video footage. Numerous automatic identification systems have been developed to solve this issue, however they are constrained to certain datasets and perform poorly when applied to different datasets. This study aims to develop an ensemble learning model utilizing a convolutional neural network (CNN) to handle deepfakes or Face2Face. We employed ensemble learning, a technique combining many classifiers to achieve higher prediction performance than a single classifier, boosting the model’s accuracy. The performance of the generated model is evaluated on Face Forensics. This work is about building a new powerful model for automatically identifying deep fake videos with the DeepFake-Detection-Challenges (DFDC) dataset. We test our model using the DFDC, one of the most difficult datasets and get an accuracy of 96%.Keywords
Cite This Article
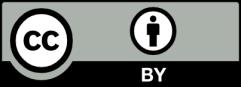