Open Access
ARTICLE
TinyML-Based Classification in an ECG Monitoring Embedded System
1 Department of Intelligence and Information, Seoul National University, Seoul, 08826, Korea
2 Nexon Korea Cop., Seongnam, Kyungki, 13487, Korea
3 Advanced CP Lab. Samsung Electronics, Suwon, Kyungki, 16677, Korea
4 Department of Electronic Engineering, Kyung Hee University, Yongin, Kyungki, 17104, Korea
5 Division of Information & Communication Engineering, Kongju National University, Cheonan, Chungcheongnam, 31080, Korea
* Corresponding Author: Yeunwoong Kyung. Email:
Computers, Materials & Continua 2023, 75(1), 1751-1764. https://doi.org/10.32604/cmc.2023.031663
Received 23 April 2022; Accepted 15 November 2022; Issue published 06 February 2023
Abstract
Recently, the development of the Internet of Things (IoT) has enabled continuous and personal electrocardiogram (ECG) monitoring. In the ECG monitoring system, classification plays an important role because it can select useful data (i.e., reduce the size of the dataset) and identify abnormal data that can be used to detect the clinical diagnosis and guide further treatment. Since the classification requires computing capability, the ECG data are usually delivered to the gateway or the server where the classification is performed based on its computing resource. However, real-time ECG data transmission continuously consumes battery and network resources, which are expensive and limited. To mitigate this problem, this paper proposes a tiny machine learning (TinyML)-based classification (i.e., TinyCES), where the ECG monitoring device performs the classification by itself based on the machine-learning model, which can reduce the memory and the network resource usages for the classification. To demonstrate the feasibility, after we configure the convolutional neural networks (CNN)-based model using ECG data from the Massachusetts Institute of Technology (MIT)-Beth Israel Hospital (BIH) arrhythmia and the Physikalisch Technische Bundesanstalt (PTB) diagnostic ECG databases, TinyCES is validated using the TinyML-supported Arduino prototype. The performance results show that TinyCES can have an approximately 97% detection ratio, which means that it has great potential to be a lightweight and resource-efficient ECG monitoring system.Keywords
Cite This Article
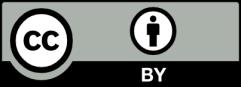