Open Access
ARTICLE
Metaheuristic Optimization of Time Series Models for Predicting Networks Traffic
1 Department of Information Technology, College of Computer and Information Sciences, Princess Nourah Bint Abdulrahman University, P.O. Box 84428, Riyadh, 11671, Saudi Arabia
2 Department of Communications and Electronics, Delta Higher Institute of Engineering and Technology, Mansoura, 35111, Egypt
3 Faculty of Artificial Intelligence, Delta University for Science and Technology, Mansoura, 35712, Egypt
4 Department of Computer Science, Faculty of Specific Education, Mansoura University, Egypt
5 Department of Computer Science, Faculty of Computer and Information Sciences, Ain Shams University, Cairo, 11566, Egypt
6 Department of Computer Science, College of Computing and Information Technology, Shaqra University, 11961, Saudi Arabia
7 Computer Engineering and Control Systems Department, Faculty of Engineering, Mansoura University, Mansoura, 35516, Egypt
8 Department of Computer Sciences, College of Computer and Information Sciences, Princess Nourah bint Abdulrahman University, P.O. Box 84428, Riyadh 11671, Saudi Arabia
* Corresponding Author: Doaa Sami Khafaga. Email:
Computers, Materials & Continua 2023, 75(1), 427-442. https://doi.org/10.32604/cmc.2023.032885
Received 01 June 2022; Accepted 05 July 2022; Issue published 06 February 2023
Abstract
Traffic prediction of wireless networks attracted many researchers and practitioners during the past decades. However, wireless traffic frequently exhibits strong nonlinearities and complicated patterns, which makes it challenging to be predicted accurately. Many of the existing approaches for predicting wireless network traffic are unable to produce accurate predictions because they lack the ability to describe the dynamic spatial-temporal correlations of wireless network traffic data. In this paper, we proposed a novel meta-heuristic optimization approach based on fitness grey wolf and dipper throated optimization algorithms for boosting the prediction accuracy of traffic volume. The proposed algorithm is employed to optimize the hyper-parameters of long short-term memory (LSTM) network as an efficient time series modeling approach which is widely used in sequence prediction tasks. To prove the superiority of the proposed algorithm, four other optimization algorithms were employed to optimize LSTM, and the results were compared. The evaluation results confirmed the effectiveness of the proposed approach in predicting the traffic of wireless networks accurately. On the other hand, a statistical analysis is performed to emphasize the stability of the proposed approach.Keywords
Cite This Article
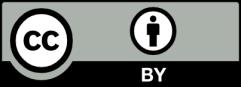