Open Access
ARTICLE
HRNetO: Human Action Recognition Using Unified Deep Features Optimization Framework
1 Department of Electrical Engineering, Riphah International University, Peshawar Rd, near Hajj Complex, I-14, Islamabad, Islamabad Capital Territory, 46000, Pakistan
2 Departmnt of Computer Science, HITEC University, Taxila, 47080, Pakistan
3 Department of Computer Science, Hanyang University, Seoul, 04763, Korea
4 Center for Computational Social Science, Hanyang University, Seoul, 04763, Korea
* Corresponding Author: Tehseen Ahsan. Email:
Computers, Materials & Continua 2023, 75(1), 1089-1105. https://doi.org/10.32604/cmc.2023.034563
Received 20 July 2022; Accepted 08 December 2022; Issue published 06 February 2023
Abstract
Human action recognition (HAR) attempts to understand a subject’s behavior and assign a label to each action performed. It is more appealing because it has a wide range of applications in computer vision, such as video surveillance and smart cities. Many attempts have been made in the literature to develop an effective and robust framework for HAR. Still, the process remains difficult and may result in reduced accuracy due to several challenges, such as similarity among actions, extraction of essential features, and reduction of irrelevant features. In this work, we proposed an end-to-end framework using deep learning and an improved tree seed optimization algorithm for accurate HAR. The proposed design consists of a few significant steps. In the first step, frame preprocessing is performed. In the second step, two pre-trained deep learning models are fine-tuned and trained through deep transfer learning using preprocessed video frames. In the next step, deep learning features of both fine-tuned models are fused using a new Parallel Standard Deviation Padding Max Value approach. The fused features are further optimized using an improved tree seed algorithm, and select the best features are finally classified by using the machine learning classifiers. The experiment was carried out on five publicly available datasets, including UT-Interaction, Weizmann, KTH, Hollywood, and IXAMS, and achieved higher accuracy than previous techniques.Keywords
Cite This Article
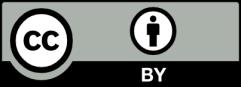