Open Access
ARTICLE
Fast Detection and Classification of Dangerous Urban Sounds Using Deep Learning
1 International Information Technology University, Almaty, Kazakhstan
2 KazNRTU named after K. I. Satbayev, Almaty, Kazakhstan
3 U. A Joldasbekov Institute of Mechanics and Engineering, Almaty, Kazakhstan
4 Faculty of Data Science and Information Technology, INTI International University, Putra Nilai, Malaysia
5 L. N. Gumilyov Eurasian National University, Astana, Kazakhstan
6 Kazakh University of Technology and Business, Astana, Kazakhstan
* Corresponding Author: Zhandos Dosbayev. Email:
Computers, Materials & Continua 2023, 75(1), 2191-2208. https://doi.org/10.32604/cmc.2023.036205
Received 20 September 2022; Accepted 23 December 2022; Issue published 06 February 2023
Abstract
Video analytics is an integral part of surveillance cameras. Compared to video analytics, audio analytics offers several benefits, including less expensive equipment and upkeep expenses. Additionally, the volume of the audio datastream is substantially lower than the video camera datastream, especially concerning real-time operating systems, which makes it less demanding of the data channel’s bandwidth needs. For instance, automatic live video streaming from the site of an explosion and gunshot to the police console using audio analytics technologies would be exceedingly helpful for urban surveillance. Technologies for audio analytics may also be used to analyze video recordings and identify occurrences. This research proposed a deep learning model based on the combination of convolutional neural network (CNN) and recurrent neural network (RNN) known as the CNN-RNN approach. The proposed model focused on automatically identifying pulse sounds that indicate critical situations in audio sources. The algorithm’s accuracy ranged from 95% to 81% when classifying noises from incidents, including gunshots, explosions, shattered glass, sirens, cries, and dog barking. The proposed approach can be applied to provide security for citizens in open and closed locations, like stadiums, underground areas, shopping malls, and other places.Keywords
Cite This Article
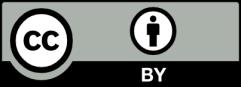