Open Access
ARTICLE
Hybrid Metaheuristics with Deep Learning Enabled Automated Deception Detection and Classification of Facial Expressions
Department of Computer Sciences, College of Computer Engineering and Sciences, Prince Sattam bin Abdulaziz University, Al Kharj, Saudi Arabia
* Corresponding Author: Haya Alaskar. Email:
Computers, Materials & Continua 2023, 75(3), 5433-5449. https://doi.org/10.32604/cmc.2023.035266
Received 14 August 2022; Accepted 08 February 2023; Issue published 29 April 2023
Abstract
Automatic deception recognition has received considerable attention from the machine learning community due to recent research on its vast application to social media, interviews, law enforcement, and the military. Video analysis-based techniques for automated deception detection have received increasing interest. This study develops a new self-adaptive population-based firefly algorithm with a deep learning-enabled automated deception detection (SAPFF-DLADD) model for analyzing facial cues. Initially, the input video is separated into a set of video frames. Then, the SAPFF-DLADD model applies the MobileNet-based feature extractor to produce a useful set of features. The long short-term memory (LSTM) model is exploited for deception detection and classification. In the final stage, the SAPFF technique is applied to optimally alter the hyperparameter values of the LSTM model, showing the novelty of the work. The experimental validation of the SAPFF-DLADD model is tested using the Miami University Deception Detection Database (MU3D), a database comprised of two classes, namely, truth and deception. An extensive comparative analysis reported a better performance of the SAPFF-DLADD model compared to recent approaches, with a higher accuracy of 99%.Keywords
Cite This Article
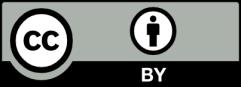