Open Access
ARTICLE
Traffic Sign Detection with Low Complexity for Intelligent Vehicles Based on Hybrid Features
1 Department of Computer Science, COMSATS University Islamabad, Wah Campus, Wah Cantt, 47040, Pakistan
2 Department of Game and Mobile Engineering, Keimyung University, Daegu, 42601, Korea
3 Department of Information and Communication Engineering, Yeungnam University, Gyeongsan, 38541, Korea
* Corresponding Authors: Jamal Hussain Shah. Email: ; Gyu Sang Choi. Email:
(This article belongs to the Special Issue: Recent Advances in Hyper Parameters Optimization, Features Optimization, and Deep Learning for Video Surveillance and Biometric Applications)
Computers, Materials & Continua 2023, 76(1), 861-879. https://doi.org/10.32604/cmc.2023.035595
Received 27 August 2022; Accepted 09 February 2023; Issue published 08 June 2023
Abstract
Globally traffic signs are used by all countries for healthier traffic flow and to protect drivers and pedestrians. Consequently, traffic signs have been of great importance for every civilized country, which makes researchers give more focus on the automatic detection of traffic signs. Detecting these traffic signs is challenging due to being in the dark, far away, partially occluded, and affected by the lighting or the presence of similar objects. An innovative traffic sign detection method for red and blue signs in color images is proposed to resolve these issues. This technique aimed to devise an efficient, robust and accurate approach. To attain this, initially, the approach presented a new formula, inspired by existing work, to enhance the image using red and green channels instead of blue, which segmented using a threshold calculated from the correlational property of the image. Next, a new set of features is proposed, motivated by existing features. Texture and color features are fused after getting extracted on the channel of Red, Green, and Blue (RGB), Hue, Saturation, and Value (HSV), and YCbCr color models of images. Later, the set of features is employed on different classification frameworks, from which quadratic support vector machine (SVM) outnumbered the others with an accuracy of 98.5%. The proposed method is tested on German Traffic Sign Detection Benchmark (GTSDB) images. The results are satisfactory when compared to the preceding work.Keywords
Cite This Article
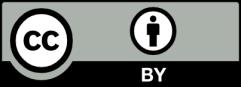