Open Access
ARTICLE
A Novel Multi-Stage Bispectral Deep Learning Method for Protein Family Classification
Biomedical Systems and Informatics Engineering Department, Hijjawi Faculty for Engineering Technology, Yarmouk University, Irbid, 21163, Jordan
* Corresponding Author: Amjed Al Fahoum. Email:
(This article belongs to the Special Issue: Intelligent Computational Models based on Machine Learning and Deep Learning for Diagnosis System)
Computers, Materials & Continua 2023, 76(1), 1173-1193. https://doi.org/10.32604/cmc.2023.038304
Received 07 December 2022; Accepted 17 April 2023; Issue published 08 June 2023
Abstract
Complex proteins are needed for many biological activities. Folding amino acid chains reveals their properties and functions. They support healthy tissue structure, physiology, and homeostasis. Precision medicine and treatments require quantitative protein identification and function. Despite technical advances and protein sequence data exploration, bioinformatics’ “basic structure” problem—the automatic deduction of a protein’s properties from its amino acid sequence—remains unsolved. Protein function inference from amino acid sequences is the main biological data challenge. This study analyzes whether raw sequencing can characterize biological facts. A massive corpus of protein sequences and the Globin-like superfamily’s related protein families generate a solid vector representation. A coding technique for each sequence in each family was devised using two representations to identify each amino acid precisely. A bispectral analysis converts encoded protein numerical sequences into images for better protein sequence and family discrimination. Training and validation employed 70% of the dataset, while 30% was used for testing. This paper examined the performance of multistage deep learning models for differentiating between sixteen protein families after encoding and representing each encoded sequence by a higher spectral representation image (Bispectrum). Cascading minimized false positive and negative cases in all phases. The initial stage focused on two classes (six groups and ten groups). The subsequent stages focused on the few classes almost accurately separated in the first stage and decreased the overlapping cases between families that appeared in single-stage deep learning classification. The single-stage technique had 64.2% +/− 22.8% accuracy, 63.3% +/− 17.1% precision, and a 63.2% +/19.4% F1-score. The two-stage technique yielded 92.2% +/− 4.9% accuracy, 92.7% +/− 7.0% precision, and a 92.3% +/− 5.0% F1-score. This work provides balanced, reliable, and precise forecasts for all families in all measures. It ensured that the new model was resilient to family variances and provided high-scoring results.Keywords
Cite This Article
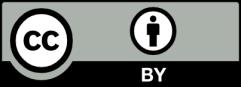