Open Access
ARTICLE
Unsupervised Anomaly Detection Approach Based on Adversarial Memory Autoencoders for Multivariate Time Series
1 Key Laboratory of Networked Control Systems, Chinese Academy of Sciences, Shenyang, 110000, China
2 Shenyang Institute of Automation, Chinese Academy of Sciences, Shenyang, 110000, China
3 Institutes for Robotics and Intelligent Manufacturing, Chinese Academy of Sciences, Shenyang, 110000, China
4 University of Chinese Academy of Sciences, Beijing, 100000, China
* Corresponding Author: Liang Jin. Email:
Computers, Materials & Continua 2023, 76(1), 329-346. https://doi.org/10.32604/cmc.2023.038595
Received 20 December 2022; Accepted 10 April 2023; Issue published 08 June 2023
Abstract
The widespread usage of Cyber Physical Systems (CPSs) generates a vast volume of time series data, and precisely determining anomalies in the data is critical for practical production. Autoencoder is the mainstream method for time series anomaly detection, and the anomaly is judged by reconstruction error. However, due to the strong generalization ability of neural networks, some abnormal samples close to normal samples may be judged as normal, which fails to detect the abnormality. In addition, the dataset rarely provides sufficient anomaly labels. This research proposes an unsupervised anomaly detection approach based on adversarial memory autoencoders for multivariate time series to solve the above problem. Firstly, an encoder encodes the input data into low-dimensional space to acquire a feature vector. Then, a memory module is used to learn the feature vector’s prototype patterns and update the feature vectors. The updating process allows partial forgetting of information to prevent model overgeneralization. After that, two decoders reconstruct the input data. Finally, this research uses the Peak Over Threshold (POT) method to calculate the threshold to determine anomalous samples from normal samples. This research uses a two-stage adversarial training strategy during model training to enlarge the gap between the reconstruction error of normal and abnormal samples. The proposed method achieves significant anomaly detection results on synthetic and real datasets from power systems, water treatment plants, and computer clusters. The F1 score reached an average of 0.9196 on the five datasets, which is 0.0769 higher than the best baseline method.Keywords
Cite This Article
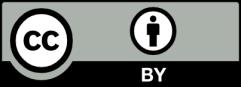