Open Access
ARTICLE
Alzheimer’s Disease Stage Classification Using a Deep Transfer Learning and Sparse Auto Encoder Method
Department of Computer Science and Engineering, SRM Institute of Science and Technology, Vadapalani, Chennai, 600026, Tamilnadu, India
* Corresponding Author: Deepthi K. Oommen. Email:
Computers, Materials & Continua 2023, 76(1), 793-811. https://doi.org/10.32604/cmc.2023.038640
Received 22 December 2022; Accepted 11 April 2023; Issue published 08 June 2023
Abstract
Alzheimer’s Disease (AD) is a progressive neurological disease. Early diagnosis of this illness using conventional methods is very challenging. Deep Learning (DL) is one of the finest solutions for improving diagnostic procedures’ performance and forecast accuracy. The disease’s widespread distribution and elevated mortality rate demonstrate its significance in the older-onset and younger-onset age groups. In light of research investigations, it is vital to consider age as one of the key criteria when choosing the subjects. The younger subjects are more susceptible to the perishable side than the older onset. The proposed investigation concentrated on the younger onset. The research used deep learning models and neuroimages to diagnose and categorize the disease at its early stages automatically. The proposed work is executed in three steps. The 3D input images must first undergo image preprocessing using Weiner filtering and Contrast Limited Adaptive Histogram Equalization (CLAHE) methods. The Transfer Learning (TL) models extract features, which are subsequently compressed using cascaded Auto Encoders (AE). The final phase entails using a Deep Neural Network (DNN) to classify the phases of AD. The model was trained and tested to classify the five stages of AD. The ensemble ResNet-18 and sparse autoencoder with DNN model achieved an accuracy of 98.54%. The method is compared to state-of-the-art approaches to validate its efficacy and performance.Keywords
Cite This Article
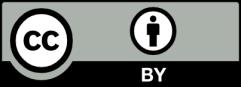