Open Access
ARTICLE
Detection of Different Stages of Alzheimer’s Disease Using CNN Classifier
1 Department of Computer Science, American International University-Bangladesh (AIUB), Dhaka, 1229, Bangladesh
2 Centre for Advanced Machine Learning and Applications (CAMLAs), Dhaka, Bangladesh
3 Department of Software Engineering, Daffodil International University, Dhaka, Bangladesh
4 Department of Computer Engineering, Umm Al-Qura University, Mecca, 24381, Saudi Arabia
5 Department of ECE, University of Saskatchewan, Saskatoon, SK S7N5A9, Canada
6 Group of Biophotomatiχ, Department of ICT, MBSTU, Santosh, Tangail, 1902, Bangladesh
* Corresponding Authors: Kawsar Ahmed. Email: ,
(This article belongs to the Special Issue: IoMT and Smart Healthcare)
Computers, Materials & Continua 2023, 76(3), 3933-3948. https://doi.org/10.32604/cmc.2023.039020
Received 08 January 2023; Accepted 13 June 2023; Issue published 08 October 2023
Abstract
Alzheimer’s disease (AD) is a neurodevelopmental impairment that results in a person’s behavior, thinking, and memory loss. The most common symptoms of AD are losing memory and early aging. In addition to these, there are several serious impacts of AD. However, the impact of AD can be mitigated by early-stage detection though it cannot be cured permanently. Early-stage detection is the most challenging task for controlling and mitigating the impact of AD. The study proposes a predictive model to detect AD in the initial phase based on machine learning and a deep learning approach to address the issue. To build a predictive model, open-source data was collected where five stages of images of AD were available as Cognitive Normal (CN), Early Mild Cognitive Impairment (EMCI), Mild Cognitive Impairment (MCI), Late Mild Cognitive Impairment (LMCI), and AD. Every stage of AD is considered as a class, and then the dataset was divided into three parts binary class, three class, and five class. In this research, we applied different preprocessing steps with augmentation techniques to efficiently identify AD. It integrates a random oversampling technique to handle the imbalance problem from target classes, mitigating the model overfitting and biases. Then three machine learning classifiers, such as random forest (RF), K-Nearest neighbor (KNN), and support vector machine (SVM), and two deep learning methods, such as convolutional neuronal network (CNN) and artificial neural network (ANN) were applied on these datasets. After analyzing the performance of the used models and the datasets, it is found that CNN with binary class outperformed 88.20% accuracy. The result of the study indicates that the model is highly potential to detect AD in the initial phase.Keywords
Cite This Article
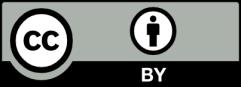