Open Access
ARTICLE
A Spider Monkey Optimization Algorithm Combining Opposition-Based Learning and Orthogonal Experimental Design
1 College of Information Science and Engineering, Jiaxing University, Jiaxing, 314001, China
2 School of Information Engineering, Huzhou University, Huzhou, 313000, China
3 Technology Research and Development Centre, Xuelong Group Co., Ltd., Ningbo, 315899, China
4 School of Internet, Jiaxing Vocational and Technical College, Jiaxing, 314036, China
* Corresponding Authors: Shigen Shen. Email: ; Helin Zhuang. Email:
Computers, Materials & Continua 2023, 76(3), 3297-3323. https://doi.org/10.32604/cmc.2023.040967
Received 06 April 2023; Accepted 29 June 2023; Issue published 08 October 2023
Abstract
As a new bionic algorithm, Spider Monkey Optimization (SMO) has been widely used in various complex optimization problems in recent years. However, the new space exploration power of SMO is limited and the diversity of the population in SMO is not abundant. Thus, this paper focuses on how to reconstruct SMO to improve its performance, and a novel spider monkey optimization algorithm with opposition-based learning and orthogonal experimental design (SMO3) is developed. A position updating method based on the historical optimal domain and particle swarm for Local Leader Phase (LLP) and Global Leader Phase (GLP) is presented to improve the diversity of the population of SMO. Moreover, an opposition-based learning strategy based on self-extremum is proposed to avoid suffering from premature convergence and getting stuck at locally optimal values. Also, a local worst individual elimination method based on orthogonal experimental design is used for helping the SMO algorithm eliminate the poor individuals in time. Furthermore, an extended SMO3 named CSMO3 is investigated to deal with constrained optimization problems. The proposed algorithm is applied to both unconstrained and constrained functions which include the CEC2006 benchmark set and three engineering problems. Experimental results show that the performance of the proposed algorithm is better than three well-known SMO algorithms and other evolutionary algorithms in unconstrained and constrained problems.Keywords
Cite This Article
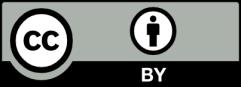