Open Access
ARTICLE
A Double-Branch Xception Architecture for Acute Hemorrhage Detection and Subtype Classification
1 Department of Computer Science, COMSATS University Islamabad, Islamabad, 45550, Pakistan
2 Department of Software Engineering, Capital University of Science & Technology, Islamabad, 44000, Pakistan
* Corresponding Author: Muhammad Imran. Email:
Computers, Materials & Continua 2023, 76(3), 3727-3744. https://doi.org/10.32604/cmc.2023.041855
Received 09 May 2023; Accepted 21 July 2023; Issue published 08 October 2023
Abstract
This study presents a deep learning model for efficient intracranial hemorrhage (ICH) detection and subtype classification on non-contrast head computed tomography (CT) images. ICH refers to bleeding in the skull, leading to the most critical life-threatening health condition requiring rapid and accurate diagnosis. It is classified as intra-axial hemorrhage (intraventricular, intraparenchymal) and extra-axial hemorrhage (subdural, epidural, subarachnoid) based on the bleeding location inside the skull. Many computer-aided diagnoses (CAD)-based schemes have been proposed for ICH detection and classification at both slice and scan levels. However, these approaches perform only binary classification and suffer from a large number of parameters, which increase storage costs. Further, the accuracy of brain hemorrhage detection in existing models is significantly low for medically critical applications. To overcome these problems, a fast and efficient system for the automatic detection of ICH is needed. We designed a double-branch model based on xception architecture that extracts spatial and instant features, concatenates them, and creates the 3D spatial context (common feature vectors) fed to a decision tree classifier for final predictions. The data employed for the experimentation was gathered during the 2019 Radiologist Society of North America (RSNA) brain hemorrhage detection challenge. Our model outperformed benchmark models and achieved better accuracy in intraventricular (99.49%), subarachnoid (99.49%), intraparenchymal (99.10%), and subdural (98.09%) categories, thereby justifying the performance of the proposed double-branch xception architecture for ICH detection and classification.Keywords
Cite This Article
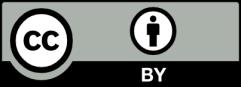