Open Access
ARTICLE
Fusion-Based Deep Learning Model for Automated Forest Fire Detection
1 Department of Natural and Applied Sciences, College of Community-Aflaj, Prince Sattam bin Abdulaziz University, Al-Kharj, 16278, Saudi Arabia
2 Department of Computer Science, College of Science & Art at Mahayil, King Khalid University, Muhayel Aseer, 62529, Saudi Arabia
3 Department of Information Technology, College of Computer, Qassim University, Al-Bukairiyah, 52571, Saudi Arabia
4 Department of Computer Sciences, College of Computer and Information Sciences, Princess Nourah bint Abdulrahman University, P.O.Box 84428, Riyadh, 11671, Saudi Arabia
5 Department of Computer and Self Development, Preparatory Year Deanship, Prince Sattam bin Abdulaziz University, Al-Kharj, 16278, Saudi Arabia
* Corresponding Author: Anwer Mustafa Hilal. Email:
Computers, Materials & Continua 2023, 77(1), 1355-1371. https://doi.org/10.32604/cmc.2023.024198
Received 08 October 2021; Accepted 09 November 2021; Issue published 31 October 2023
Abstract
Earth resource and environmental monitoring are essential areas that can be used to investigate the environmental conditions and natural resources supporting sustainable policy development, regulatory measures, and their implementation elevating the environment. Large-scale forest fire is considered a major harmful hazard that affects climate change and life over the globe. Therefore, the early identification of forest fires using automated tools is essential to avoid the spread of fire to a large extent. Therefore, this paper focuses on the design of automated forest fire detection using a fusion-based deep learning (AFFD-FDL) model for environmental monitoring. The AFFD-FDL technique involves the design of an entropy-based fusion model for feature extraction. The combination of the handcrafted features using histogram of gradients (HOG) with deep features using SqueezeNet and Inception v3 models. Besides, an optimal extreme learning machine (ELM) based classifier is used to identify the existence of fire or not. In order to properly tune the parameters of the ELM model, the oppositional glowworm swarm optimization (OGSO) algorithm is employed and thereby improves the forest fire detection performance. A wide range of simulation analyses takes place on a benchmark dataset and the results are inspected under several aspects. The experimental results highlighted the betterment of the AFFD-FDL technique over the recent state of art techniques.Keywords
Cite This Article
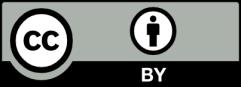