Open Access
ARTICLE
Micro-Expression Recognition Based on Spatio-Temporal Feature Extraction of Key Regions
1 College of Computer Science, Hunan University of Technology, Zhuzhou, 412007, China
2 Intelligent Information Perception and Processing Technology, Hunan Province Key Laboratory, Zhuzhou, 412007, China
* Corresponding Author: Qiang Liu. Email:
Computers, Materials & Continua 2023, 77(1), 1373-1392. https://doi.org/10.32604/cmc.2023.037216
Received 27 October 2022; Accepted 03 March 2023; Issue published 31 October 2023
Abstract
Aiming at the problems of short duration, low intensity, and difficult detection of micro-expressions (MEs), the global and local features of ME video frames are extracted by combining spatial feature extraction and temporal feature extraction. Based on traditional convolution neural network (CNN) and long short-term memory (LSTM), a recognition method combining global identification attention network (GIA), block identification attention network (BIA) and bi-directional long short-term memory (Bi-LSTM) is proposed. In the BIA, the ME video frame will be cropped, and the training will be carried out by cropping into 24 identification blocks (IBs), 10 IBs and uncropped IBs. To alleviate the overfitting problem in training, we first extract the basic features of the pre-processed sequence through the transfer learning layer, and then extract the global and local spatial features of the output data through the GIA layer and the BIA layer, respectively. In the BIA layer, the input data will be cropped into local feature vectors with attention weights to extract the local features of the ME frames; in the GIA layer, the global features of the ME frames will be extracted. Finally, after fusing the global and local feature vectors, the ME time-series information is extracted by Bi-LSTM. The experimental results show that using IBs can significantly improve the model's ability to extract subtle facial features, and the model works best when 10 IBs are used.Keywords
Cite This Article
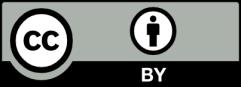