Open Access
ARTICLE
Unweighted Voting Method to Detect Sinkhole Attack in RPL-Based Internet of Things Networks
1 National Advanced IPv6 Centre (NAv6), University Sains Malaysia, Gelugor, Penang, 11800, Malaysia
2 Applied College, Najran University, King Abdulaziz Street, P.O. Box 1988, Najran, Saudi Arabia
3 Cybersecurity Department, School of Information Technology, American University of Madaba (AUM), Amman, 11821, Jordan
* Corresponding Author: Mohammed Anbar. Email:
Computers, Materials & Continua 2023, 77(1), 491-515. https://doi.org/10.32604/cmc.2023.041108
Received 11 April 2023; Accepted 09 August 2023; Issue published 31 October 2023
Abstract
The Internet of Things (IoT) consists of interconnected smart devices communicating and collecting data. The Routing Protocol for Low-Power and Lossy Networks (RPL) is the standard protocol for Internet Protocol Version 6 (IPv6) in the IoT. However, RPL is vulnerable to various attacks, including the sinkhole attack, which disrupts the network by manipulating routing information. This paper proposes the Unweighted Voting Method (UVM) for sinkhole node identification, utilizing three key behavioral indicators: DODAG Information Object (DIO) Transaction Frequency, Rank Harmony, and Power Consumption. These indicators have been carefully selected based on their contribution to sinkhole attack detection and other relevant features used in previous research. The UVM method employs an unweighted voting mechanism, where each voter or rule holds equal weight in detecting the presence of a sinkhole attack based on the proposed indicators. The effectiveness of the UVM method is evaluated using the COOJA simulator and compared with existing approaches. Notably, the proposed approach fulfills power consumption requirements for constrained nodes without increasing consumption due to the deployment design. In terms of detection accuracy, simulation results demonstrate a high detection rate ranging from 90% to 100%, with a low false-positive rate of 0% to 0.2%. Consequently, the proposed approach surpasses Ensemble Learning Intrusion Detection Systems by leveraging three indicators and three supporting rules.Keywords
Cite This Article
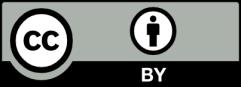