Open Access
ARTICLE
Automated Pavement Crack Detection Using Deep Feature Selection and Whale Optimization Algorithm
Faculty of Engineering, China University of Geosciences, Wuhan, 430074, China
* Corresponding Author: Li Wu. Email:
(This article belongs to the Special Issue: Machine Vision Detection and Intelligent Recognition)
Computers, Materials & Continua 2023, 77(1), 63-77. https://doi.org/10.32604/cmc.2023.042183
Received 22 May 2023; Accepted 29 August 2023; Issue published 31 October 2023
Abstract
Pavement crack detection plays a crucial role in ensuring road safety and reducing maintenance expenses. Recent advancements in deep learning (DL) techniques have shown promising results in detecting pavement cracks; however, the selection of relevant features for classification remains challenging. In this study, we propose a new approach for pavement crack detection that integrates deep learning for feature extraction, the whale optimization algorithm (WOA) for feature selection, and random forest (RF) for classification. The performance of the models was evaluated using accuracy, recall, precision, F1 score, and area under the receiver operating characteristic curve (AUC). Our findings reveal that Model 2, which incorporates RF into the ResNet-18 architecture, outperforms baseline Model 1 across all evaluation metrics. Nevertheless, our proposed model, which combines ResNet-18 with both WOA and RF, achieves significantly higher accuracy, recall, precision, and F1 score compared to the other two models. These results underscore the effectiveness of integrating RF and WOA into ResNet-18 for pavement crack detection applications. We applied the proposed approach to a dataset of pavement images, achieving an accuracy of 97.16% and an AUC of 0.984. Our results demonstrate that the proposed approach surpasses existing methods for pavement crack detection, offering a promising solution for the automatic identification of pavement cracks. By leveraging this approach, potential safety hazards can be identified more effectively, enabling timely repairs and maintenance measures. Lastly, the findings of this study also emphasize the potential of integrating RF and WOA with deep learning for pavement crack detection, providing road authorities with the necessary tools to make informed decisions regarding road infrastructure maintenance.Keywords
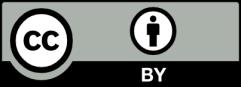