Open Access
ARTICLE
Application of the Deep Convolutional Neural Network for the Classification of Auto Immune Diseases
1 Department of Computer Science and Information Technology, Sarhad University of Science and Information
Technology, Peshawar, 25000, Pakistan
2 Department of Computer Science, University of Engineering and Technology, Mardan, 23200, Pakistan
3 EIAS Data Science and Blockchain Lab, College of Computer and Information Sciences, Prince Sultan University,
Riyadh, 11586, Saudi Arabia
* Corresponding Author: Gauhar Ali. Email:
(This article belongs to the Special Issue: Emerging Trends, Advances and Challenges of IoT in Healthcare and Education)
Computers, Materials & Continua 2023, 77(1), 647-664. https://doi.org/10.32604/cmc.2023.038748
Received 27 December 2022; Accepted 30 May 2023; Issue published 31 October 2023
Abstract
IIF (Indirect Immune Florescence) has gained much attention recently due to its importance in medical sciences. The primary purpose of this work is to highlight a step-by-step methodology for detecting autoimmune diseases. The use of IIF for detecting autoimmune diseases is widespread in different medical areas. Nearly 80 different types of autoimmune diseases have existed in various body parts. The IIF has been used for image classification in both ways, manually and by using the Computer-Aided Detection (CAD) system. The data scientists conducted various research works using an automatic CAD system with low accuracy. The diseases in the human body can be detected with the help of Transfer Learning (TL), an advanced Convolutional Neural Network (CNN) approach. The baseline paper applied the manual classification to the MIVIA dataset of Human Epithelial cells (HEP) type II cells and the Sub Class Discriminant (SDA) analysis technique used to detect autoimmune diseases. The technique yielded an accuracy of up to 90.03%, which was not reliable for detecting autoimmune disease in the mitotic cells of the body. In the current research, the work has been performed on the MIVIA data set of HEP type II cells by using four well-known models of TL. Data augmentation and normalization have been applied to the dataset to overcome the problem of overfitting and are also used to improve the performance of TL models. These models are named Inception V3, Dens Net 121, VGG-16, and Mobile Net, and their performance can be calculated through parameters of the confusion matrix (accuracy, precision, recall, and F1 measures). The results show that the accuracy value of VGG- 16 is 78.00%, Inception V3 is 92.00%, Dense Net 121 is 95.00%, and Mobile Net shows 88.00% accuracy, respectively. Therefore, DenseNet-121 shows the highest performance with suitable analysis of autoimmune diseases. The overall performance highlighted that TL is a suitable and enhanced technique compared to its counterparts. Also, the proposed technique is used to detect autoimmune diseases with a minimal margin of errors and flaws.Keywords
Cite This Article
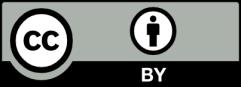