Open Access
ARTICLE
Machine Learning Design of Aluminum-Lithium Alloys with High Strength
1
College of Semiconductors and Physics, North University of China, Taiyuan, 030051, China
2
School of Materials Science and Engineering, Collaborative Innovation Center of Ministry of Education and Shanxi Province for
High-Performance Al/Mg Alloy Materials, North University of China, Taiyuan, 030051, China
3
Beijing Advanced Innovation Center for Materials Genome Engineering, University of Science and Technology Beijing, Beijing,
100083, China
4
Institute of Materials Intelligent Technology, Liaoning Academy of Materials, Shenyang, 110004, China
* Corresponding Authors: Yongmei Zhang. Email: ; Yuhong Zhao. Email:
Computers, Materials & Continua 2023, 77(2), 1393-1409. https://doi.org/10.32604/cmc.2023.045871
Received 10 September 2023; Accepted 19 October 2023; Issue published 29 November 2023
Abstract
Due to the large unexplored compositional space, long development cycle, and high cost of traditional trial-anderror experiments, designing high strength aluminum-lithium alloys is a great challenge. This work establishes a performance-oriented machine learning design strategy for aluminum-lithium alloys to simplify and shorten the development cycle. The calculation results indicate that radial basis function (RBF) neural networks exhibit better predictive ability than back propagation (BP) neural networks. The RBF neural network predicted tensile and yield strengths with determination coefficients of 0.90 and 0.96, root mean square errors of 30.68 and 25.30, and mean absolute errors of 28.15 and 19.08, respectively. In the validation experiment, the comparison between experimental data and predicted data demonstrated the robustness of the two neural network models. The tensile and yield strengths of Al-2Li-1Cu-3Mg-0.2Zr (wt.%) alloy are 17.8 and 3.5 MPa higher than those of the Al-1Li- 4.5Cu-0.2Zr (wt.%) alloy, which has the best overall performance, respectively. It demonstrates the reliability of the neural network model in designing high strength aluminum-lithium alloys, which provides a way to improve research and development efficiency.Keywords
Cite This Article
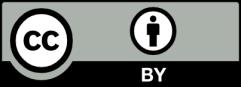