Open Access
REVIEW
Ensuring User Privacy and Model Security via Machine Unlearning: A Review
1 College of Computer, National University of Defense Technology, Changsha, 410073, China
2 School of Computing and Communications, Lancaster University, England, B23, UK
* Corresponding Author: Zhiping Cai. Email:
Computers, Materials & Continua 2023, 77(2), 2645-2656. https://doi.org/10.32604/cmc.2023.032307
Received 16 January 2021; Accepted 30 October 2021; Issue published 29 November 2023
Abstract
As an emerging discipline, machine learning has been widely used in artificial intelligence, education, meteorology and other fields. In the training of machine learning models, trainers need to use a large amount of practical data, which inevitably involves user privacy. Besides, by polluting the training data, a malicious adversary can poison the model, thus compromising model security. The data provider hopes that the model trainer can prove to them the confidentiality of the model. Trainer will be required to withdraw data when the trust collapses. In the meantime, trainers hope to forget the injected data to regain security when finding crafted poisoned data after the model training. Therefore, we focus on forgetting systems, the process of which we call machine unlearning, capable of forgetting specific data entirely and efficiently. In this paper, we present the first comprehensive survey of this realm. We summarize and categorize existing machine unlearning methods based on their characteristics and analyze the relation between machine unlearning and relevant fields (e.g., inference attacks and data poisoning attacks). Finally, we briefly conclude the existing research directions.Keywords
Cite This Article
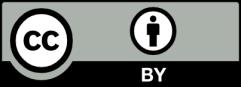