Open Access
ARTICLE
A Novel Unsupervised MRI Synthetic CT Image Generation Framework with Registration Network
1 Heilongjiang Provincial Key Laboratory of Complex Intelligent System and Integration, School of Automation, Harbin University of Science and Technology, Harbin, 150080, China
2 Institute for Brain Research and Rehabilitation, South China Normal University, Guangzhou, 510631, China
3 Department of Radiation Oncology, Sun Yat-sen University Cancer Center, State Key Laboratory of Oncology in South China, Collaborative Innovation Center for Cancer Medicine, Guangdong Key Laboratory of Nasopharyngeal Carcinoma Diagnosis and Therapy, Guangzhou, 510060, China
* Corresponding Author: Xin Yang. Email:
Computers, Materials & Continua 2023, 77(2), 2271-2287. https://doi.org/10.32604/cmc.2023.039062
Received 09 January 2023; Accepted 01 June 2023; Issue published 29 November 2023
Abstract
In recent years, radiotherapy based only on Magnetic Resonance (MR) images has become a hot spot for radiotherapy planning research in the current medical field. However, functional computed tomography (CT) is still needed for dose calculation in the clinic. Recent deep-learning approaches to synthesized CT images from MR images have raised much research interest, making radiotherapy based only on MR images possible. In this paper, we proposed a novel unsupervised image synthesis framework with registration networks. This paper aims to enforce the constraints between the reconstructed image and the input image by registering the reconstructed image with the input image and registering the cycle-consistent image with the input image. Furthermore, this paper added ConvNeXt blocks to the network and used large kernel convolutional layers to improve the network’s ability to extract features. This research used the collected head and neck data of 180 patients with nasopharyngeal carcinoma to experiment and evaluate the training model with four evaluation metrics. At the same time, this research made a quantitative comparison of several commonly used model frameworks. We evaluate the model performance in four evaluation metrics which achieve Mean Absolute Error (MAE), Root Mean Square Error (RMSE), Peak Signal-to-Noise Ratio (PSNR), and Structural Similarity (SSIM) are 18.55 ± 1.44, 86.91 ± 4.31, 33.45 ± 0.74 and 0.960 ± 0.005, respectively. Compared with other methods, MAE decreased by 2.17, RMSE decreased by 7.82, PSNR increased by 0.76, and SSIM increased by 0.011. The results show that the model proposed in this paper outperforms other methods in the quality of image synthesis. The work in this paper is of guiding significance to the study of MR-only radiotherapy planning.Keywords
Cite This Article
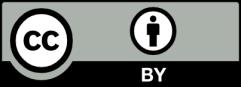