Open Access
ARTICLE
A Memory-Guided Anomaly Detection Model with Contrastive Learning for Multivariate Time Series
1 School of Electronic and Information Engineering, Hebei University of Technology, Tianjin, 300401, China
2 School of Artificial Intelligence, Hebei University of Technology, Tianjin, 300401, China
3 School of Computer Science and Engineering, University of Electronic Science and Technology of China, Chengdu, 611731, China
* Corresponding Author: Ping He. Email:
(This article belongs to the Special Issue: Development and Industrial Application of AI Technologies)
Computers, Materials & Continua 2023, 77(2), 1893-1910. https://doi.org/10.32604/cmc.2023.044253
Received 25 July 2023; Accepted 25 September 2023; Issue published 29 November 2023
Abstract
Some reconstruction-based anomaly detection models in multivariate time series have brought impressive performance advancements but suffer from weak generalization ability and a lack of anomaly identification. These limitations can result in the misjudgment of models, leading to a degradation in overall detection performance. This paper proposes a novel transformer-like anomaly detection model adopting a contrastive learning module and a memory block (CLME) to overcome the above limitations. The contrastive learning module tailored for time series data can learn the contextual relationships to generate temporal fine-grained representations. The memory block can record normal patterns of these representations through the utilization of attention-based addressing and reintegration mechanisms. These two modules together effectively alleviate the problem of generalization. Furthermore, this paper introduces a fusion anomaly detection strategy that comprehensively takes into account the residual and feature spaces. Such a strategy can enlarge the discrepancies between normal and abnormal data, which is more conducive to anomaly identification. The proposed CLME model not only efficiently enhances the generalization performance but also improves the ability of anomaly detection. To validate the efficacy of the proposed approach, extensive experiments are conducted on well-established benchmark datasets, including SWaT, PSM, WADI, and MSL. The results demonstrate outstanding performance, with F1 scores of 90.58%, 94.83%, 91.58%, and 91.75%, respectively. These findings affirm the superiority of the CLME model over existing state-of-the-art anomaly detection methodologies in terms of its ability to detect anomalies within complex datasets accurately.Keywords
Cite This Article
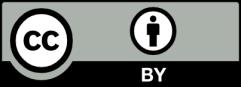