Open Access
ARTICLE
Classification of Electrocardiogram Signals for Arrhythmia Detection Using Convolutional Neural Network
1 Department of Computer Science and IT, Lahore Lead University, Lahore, 54000, Pakistan
2 Department of Information Sciences, Division of Science and Technology, University of Education, Lahore, 54000, Pakistan
3 Faculty of Computing and Informatics, University Malaysia Sabah, Jalan UMS, Kota Kinabalu, Sabah, 88400, Malaysia
4 Computer Science Department UBIT, Karachi University, Karachi, 75270, Pakistan
5 Department of Basic Sciences, Deanship of Common First Year, Jouf University, Sakaka, Aljouf, 72341, Saudi Arabia
* Corresponding Author: Ag. Asri Ag. Ibrahim. Email:
Computers, Materials & Continua 2023, 77(3), 3817-3834. https://doi.org/10.32604/cmc.2023.032275
Received 12 May 2022; Accepted 30 January 2023; Issue published 26 December 2023
Abstract
With the help of computer-aided diagnostic systems, cardiovascular diseases can be identified timely manner to minimize the mortality rate of patients suffering from cardiac disease. However, the early diagnosis of cardiac arrhythmia is one of the most challenging tasks. The manual analysis of electrocardiogram (ECG) data with the help of the Holter monitor is challenging. Currently, the Convolutional Neural Network (CNN) is receiving considerable attention from researchers for automatically identifying ECG signals. This paper proposes a 9-layer-based CNN model to classify the ECG signals into five primary categories according to the American National Standards Institute (ANSI) standards and the Association for the Advancement of Medical Instruments (AAMI). The Massachusetts Institute of Technology-Beth Israel Hospital (MIT-BIH) arrhythmia dataset is used for the experiment. The proposed model outperformed the previous model in terms of accuracy and achieved a sensitivity of 99.0% and a positivity predictively 99.2% in the detection of a Ventricular Ectopic Beat (VEB). Moreover, it also gained a sensitivity of 99.0% and positivity predictively of 99.2% for the detection of a supraventricular ectopic beat (SVEB). The overall accuracy of the proposed model is 99.68%.Keywords
Cite This Article
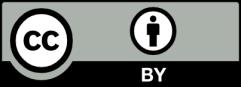