Open Access
ARTICLE
Nuclei Segmentation in Histopathology Images Using Structure-Preserving Color Normalization Based Ensemble Deep Learning Frameworks
1 Centre for Cyber-Physical Systems, Vellore Institute of Technology, Chennai, 600127, India
2 School of Computer Science and Engineering, Vellore Institute of Technology, Chennai, 600127, India
3 Centre for Advanced Data Science, Vellore Institute of Technology, Chennai, 600127, India
* Corresponding Author: Sandeep Kumar Satapathy. Email:
Computers, Materials & Continua 2023, 77(3), 3077-3094. https://doi.org/10.32604/cmc.2023.042718
Received 09 June 2023; Accepted 14 September 2023; Issue published 26 December 2023
Abstract
This paper presents a novel computerized technique for the segmentation of nuclei in hematoxylin and eosin (H&E) stained histopathology images. The purpose of this study is to overcome the challenges faced in automated nuclei segmentation due to the diversity of nuclei structures that arise from differences in tissue types and staining protocols, as well as the segmentation of variable-sized and overlapping nuclei. To this extent, the approach proposed in this study uses an ensemble of the UNet architecture with various Convolutional Neural Networks (CNN) architectures as encoder backbones, along with stain normalization and test time augmentation, to improve segmentation accuracy. Additionally, this paper employs a Structure-Preserving Color Normalization (SPCN) technique as a preprocessing step for stain normalization. The proposed model was trained and tested on both single-organ and multi-organ datasets, yielding an F1 score of 84.11%, mean Intersection over Union (IoU) of 81.67%, dice score of 84.11%, accuracy of 92.58% and precision of 83.78% on the multi-organ dataset, and an F1 score of 87.04%, mean IoU of 86.66%, dice score of 87.04%, accuracy of 96.69% and precision of 87.57% on the single-organ dataset. These findings demonstrate that the proposed model ensemble coupled with the right pre-processing and post-processing techniques enhances nuclei segmentation capabilities.Keywords
Cite This Article
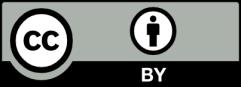