Open Access
ARTICLE
YOLO-DD: Improved YOLOv5 for Defect Detection
1 School of Electronic and Information Engineering, Foshan University, Foshan, 528000, China
2 School of Mechatronic Engineering and Automation, Foshan University, Foshan, 528000, China
* Corresponding Author: Lufeng Luo. Email:
Computers, Materials & Continua 2024, 78(1), 759-780. https://doi.org/10.32604/cmc.2023.041600
Received 28 April 2023; Accepted 29 July 2023; Issue published 30 January 2024
Abstract
As computer technology continues to advance, factories have increasingly higher demands for detecting defects. However, detecting defects in a plant environment remains a challenging task due to the presence of complex backgrounds and defects of varying shapes and sizes. To address this issue, this paper proposes YOLO-DD, a defect detection model based on YOLOv5 that is effective and robust. To improve the feature extraction process and better capture global information, the vanilla YOLOv5 is augmented with a new module called Relative-Distance-Aware Transformer (RDAT). Additionally, an Information Gap Filling Strategy (IGFS) is proposed to improve the fusion of features at different scales. The classic lightweight attention mechanism Squeeze-and-Excitation (SE) module is also incorporated into the neck section to enhance feature expression and improve the model’s performance. Experimental results on the NEU-DET dataset demonstrate that YOLO-DD achieves competitive results compared to state-of-the-art methods, with a 2.0% increase in accuracy compared to the original YOLOv5, achieving 82.41% accuracy and 38.25 FPS (frames per second). The model is also tested on a self-constructed fabric defect dataset, and the results show that YOLO-DD is more stable and has higher accuracy than the original YOLOv5, demonstrating its stability and generalization ability. The high efficiency of YOLO-DD enables it to meet the requirements of industrial high accuracy and real-time detection.Keywords
Cite This Article
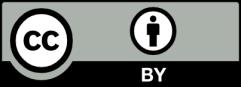