Open Access
ARTICLE
Network Configuration Entity Extraction Method Based on Transformer with Multi-Head Attention Mechanism
1 State Key Laboratory of Networking and Switching Technology, Beijing University of Posts and Telecommunications, Beijing, 100876, China
2 Product Development Department, China Unicom Smart City Research Institute, Beijing, 100044, China
* Corresponding Author: Zhipeng Gao. Email:
(This article belongs to the Special Issue: Recognition Tasks with Transformers)
Computers, Materials & Continua 2024, 78(1), 735-757. https://doi.org/10.32604/cmc.2023.045807
Received 08 September 2023; Accepted 01 November 2023; Issue published 30 January 2024
Abstract
Nowadays, ensuring the quality of network services has become increasingly vital. Experts are turning to knowledge graph technology, with a significant emphasis on entity extraction in the identification of device configurations. This research paper presents a novel entity extraction method that leverages a combination of active learning and attention mechanisms. Initially, an improved active learning approach is employed to select the most valuable unlabeled samples, which are subsequently submitted for expert labeling. This approach successfully addresses the problems of isolated points and sample redundancy within the network configuration sample set. Then the labeled samples are utilized to train the model for network configuration entity extraction. Furthermore, the multi-head self-attention of the transformer model is enhanced by introducing the Adaptive Weighting method based on the Laplace mixture distribution. This enhancement enables the transformer model to dynamically adapt its focus to words in various positions, displaying exceptional adaptability to abnormal data and further elevating the accuracy of the proposed model. Through comparisons with Random Sampling (RANDOM), Maximum Normalized Log-Probability (MNLP), Least Confidence (LC), Token Entrop (TE), and Entropy Query by Bagging (EQB), the proposed method, Entropy Query by Bagging and Maximum Influence Active Learning (EQBMIAL), achieves comparable performance with only 40% of the samples on both datasets, while other algorithms require 50% of the samples. Furthermore, the entity extraction algorithm with the Adaptive Weighted Multi-head Attention mechanism (AW-MHA) is compared with BILSTM-CRF, Mutil_Attention-Bilstm-Crf, Deep_Neural_Model_NER and BERT_Transformer, achieving precision rates of 75.98% and 98.32% on the two datasets, respectively. Statistical tests demonstrate the statistical significance and effectiveness of the proposed algorithms in this paper.Keywords
Cite This Article
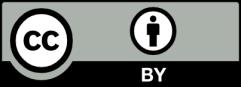