Open Access
ARTICLE
Recommendation Method for Contrastive Enhancement of Neighborhood Information
School of Computer Science and Engineering, North Minzu University, Ningxia, 750021, China
* Corresponding Author: Beijing Zhou. Email:
Computers, Materials & Continua 2024, 78(1), 453-472. https://doi.org/10.32604/cmc.2023.046560
Received 07 October 2023; Accepted 14 November 2023; Issue published 30 January 2024
Abstract
Knowledge graph can assist in improving recommendation performance and is widely applied in various personalized recommendation domains. However, existing knowledge-aware recommendation methods face challenges such as weak user-item interaction supervisory signals and noise in the knowledge graph. To tackle these issues, this paper proposes a neighbor information contrast-enhanced recommendation method by adding subtle noise to construct contrast views and employing contrastive learning to strengthen supervisory signals and reduce knowledge noise. Specifically, first, this paper adopts heterogeneous propagation and knowledge-aware attention networks to obtain multi-order neighbor embedding of users and items, mining the high-order neighbor information of users and items. Next, in the neighbor information, this paper introduces weak noise following a uniform distribution to construct neighbor contrast views, effectively reducing the time overhead of view construction. This paper then performs contrastive learning between neighbor views to promote the uniformity of view information, adjusting the neighbor structure, and achieving the goal of reducing the knowledge noise in the knowledge graph. Finally, this paper introduces multi-task learning to mitigate the problem of weak supervisory signals. To validate the effectiveness of our method, experiments are conducted on the MovieLens-1M, MovieLens-20M, Book-Crossing, and Last-FM datasets. The results show that compared to the best baselines, our method shows significant improvements in AUC and F1.Keywords
Cite This Article
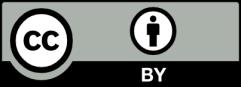