Open Access
ARTICLE
Lightweight Intrusion Detection Using Reservoir Computing
1 Joint Laboratory on Cyberspace Security, China Southern Power Grid, Guangzhou, 510080, China
2 College of Cyber Security, Jinan University, Guangzhou, 510632, China
3 Guangdong Power Grid Company Limited, Guangzhou, 510663, China
4 School of Computer Science and Technology, Guangdong University of Technology, Guangzhou, 510006, China
5 China Southern Power Grid, Extra High Voltage Transmission Company, Guangzhou, China
* Corresponding Author: Shanxiang Lyu. Email:
(This article belongs to the Special Issue: Security and Privacy for Blockchain-empowered Internet of Things)
Computers, Materials & Continua 2024, 78(1), 1345-1361. https://doi.org/10.32604/cmc.2023.047079
Received 24 October 2023; Accepted 30 November 2023; Issue published 30 January 2024
Abstract
The blockchain-empowered Internet of Vehicles (IoV) enables various services and achieves data security and privacy, significantly advancing modern vehicle systems. However, the increased frequency of data transmission and complex network connections among nodes also make them more susceptible to adversarial attacks. As a result, an efficient intrusion detection system (IDS) becomes crucial for securing the IoV environment. Existing IDSs based on convolutional neural networks (CNN) often suffer from high training time and storage requirements. In this paper, we propose a lightweight IDS solution to protect IoV against both intra-vehicle and external threats. Our approach achieves superior performance, as demonstrated by key metrics such as accuracy and precision. Specifically, our method achieves accuracy rates ranging from 99.08% to 100% on the Car-Hacking dataset, with a remarkably short training time.Keywords
Cite This Article
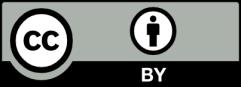